Spot Detection#
Last updated May 31, 2023
In this section we will build a model that will detect beetle appendages in images of beetles. The training data is courtesy of the Parker lab at Caltech. The images of ants are annotated with coordinates for different types of appendages (head, abdomen, thorax, etc.)
!pip install tensorflow-addons "deepcell==0.9.0"
import os
import glob
import imageio
import skimage
import skimage.exposure
import skimage.transform
import copy
import re
import tensorflow as tf
import tensorflow_addons as tfa
import numpy as np
import pandas as pd
import matplotlib.pyplot as plt
import sklearn.model_selection
from tqdm.notebook import tqdm
To solve this problem, we will treat it as a regression problem. For each image we will try to predict a transform image. This transform image will tell us how far each pixel is from the nearest spot. By looking for the extrema in the predicted transform image, we will be able to identify the appendages.
The transform we will use is
where $\alpha$ is a parameter that determines the length scale. We will set $\alpha$ to be ~10 pixels, which is roughly the length scale for an appendage.
Load data#
!wget https://storage.googleapis.com/datasets-spring2021/bugs.npz
!wget https://storage.googleapis.com/datasets-spring2021/bug_annotations.csv
# Load data and convert annotations to transform images
from scipy.ndimage.morphology import distance_transform_edt
# We will compute the transforms for each set of annotations using the
# coord_to_dist function
def coord_to_dist(point_list,
image_shape=(512,512),
alpha=10,
dy=1,
dx=1):
# create an image with 0 = pixel containing point
# from point_list, 1 = pixel not containing point from point_list
contains_point = np.ones(image_shape)
for ind, [x, y] in enumerate(point_list):
nearest_pixel_y_ind = int(round(y/dy))
nearest_pixel_x_ind = int(round(x/dx))
contains_point[nearest_pixel_y_ind, nearest_pixel_x_ind] = 0
edt, inds = distance_transform_edt(contains_point, return_indices=True, sampling=[dy, dx])
transform = 1/(1+edt/alpha)
return transform
def load_bug_data():
bugs_path = 'bugs.npz'
csv_path = 'bug_annotations.csv'
bug_file = np.load(bugs_path)
bug_imgs = bug_file['X'].astype('float')
bug_imgs /= 255.0
print(bug_imgs.shape)
# Normalize bug images
bug_imgs_norm = []
for i in tqdm(range(bug_imgs.shape[0])):
img = bug_imgs[i,...]
img = skimage.exposure.equalize_adapthist(img)
img = skimage.exposure.rescale_intensity(img, out_range=(0,1))
img = skimage.transform.resize(img, (256,256))
bug_imgs_norm.append(img)
bug_imgs = np.stack(bug_imgs_norm, axis=0)
bug_imgs = np.expand_dims(bug_imgs, axis=-1)
# Load annotations
csv_df = pd.read_csv(csv_path)
csv_df.head()
head_list = []
thorax_list = []
abdomen_list = []
# Convert point_lists to transform images
for i in tqdm(range(bug_imgs.shape[0])):
# Load the annotation for the image
ann = csv_df.loc[csv_df['fileindex']==i]
head_ann = ann.loc[ann['bodyparts']=='head'][['x', 'y']] * 256/500
thorax_ann = ann.loc[ann['bodyparts']=='thorax'][['x', 'y']] * 256/500
abdomen_ann = ann.loc[ann['bodyparts']=='abdomen'][['x', 'y']] * 256/500
head_ann = np.array(head_ann)
thorax_ann = np.array(thorax_ann)
abdomen_ann = np.array(abdomen_ann)
# Compute transforms
head_distance_img = coord_to_dist(head_ann, image_shape=(256,256))
thorax_distance_img = coord_to_dist(thorax_ann, image_shape=(256,256))
abdomen_distance_img = coord_to_dist(abdomen_ann, image_shape=(256,256))
head_list.append(head_distance_img)
thorax_list.append(thorax_distance_img)
abdomen_list.append(abdomen_distance_img)
head_distance = np.stack(head_list, axis=0)
thorax_distance = np.stack(thorax_list, axis=0)
abdomen_distance = np.stack(abdomen_list, axis=0)
head_distance = np.expand_dims(head_distance, axis=-1)
thorax_distance = np.expand_dims(thorax_distance, axis=-1)
abdomen_distance = np.expand_dims(abdomen_distance, axis=-1)
return bug_imgs, head_distance, thorax_distance, abdomen_distance
bug_imgs, head_distance, thorax_distance, abdomen_distance = load_bug_data()
(847, 500, 500)
# Visually inspect the images and transforms to make sure they are correct
print(bug_imgs.shape)
csv_path = 'bug_annotations.csv'
csv_df = pd.read_csv(csv_path)
fig, axes = plt.subplots(1,4,figsize=(20,20))
index = 0
ann = csv_df.loc[csv_df['fileindex']==index]
head_ann = np.array(ann.loc[ann['bodyparts']=='head'][['y', 'x']]) * 256/500
thorax_ann = np.array(ann.loc[ann['bodyparts']=='thorax'][['y', 'x']]) * 256/500
abdomen_ann = np.array(ann.loc[ann['bodyparts']=='abdomen'][['y', 'x']]) * 256/500
axes[0].imshow(bug_imgs[index,...], cmap='gray')
axes[0].scatter(head_ann[:,1], head_ann[:,0], color='c')
axes[0].scatter(thorax_ann[:,1], thorax_ann[:,0], color='m')
axes[0].scatter(abdomen_ann[:,1], abdomen_ann[:,0], color='y')
axes[1].imshow(head_distance[index,:,:], cmap='gray')
axes[1].scatter(head_ann[:,1], head_ann[:,0], color='c')
axes[2].imshow(thorax_distance[index,:,:], cmap='gray')
axes[2].scatter(thorax_ann[:,1], thorax_ann[:,0], color='m')
axes[3].imshow(abdomen_distance[index,:,:], cmap='gray')
axes[3].scatter(abdomen_ann[:,1], abdomen_ann[:,0], color='y')
(847, 256, 256, 1)
<matplotlib.collections.PathCollection at 0x7fa9ca535668>
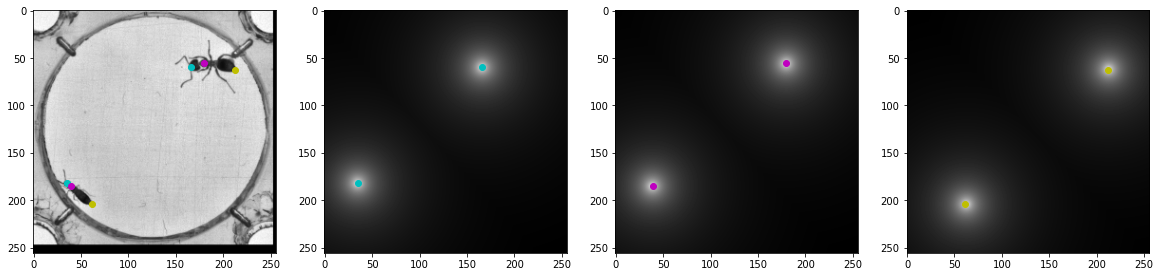
Prepare dataset object#
Creating the dataset object is more challenging for this problem due to our problem framing. Because we are predicting the transform images, we need to make sure we apply the same transform to the raw image and the transform images. If we do not, then the information content of the transform images (e.g., where the appendages are) will be lost.
Doing this using tensorflow dataset objects is a little challenging. We will need to specify the augmentation operation that will be applied, and then specifically apply it to each of image (e.g., the raw image and each of the transform images). To specify the augmentation operation, we will need to specify the transform matrix. Moreover, this specification needs to be done with tensorflow objects (e.g., tensorflow tensors and operations from tf.image). These steps are executed in the following cell.
An additional practical programming note - because we are predicting 3 transforms, we will need a network that produces 3 prediction images. It can get confusing to keep track of which prediction image is which. To mitigate this, we will have our dataset object produce dictionaries rather than tuples or lists. The key names in the dictionary will match the names of the corresponding layers in the deep learning model. This will help us keep track of which transform image is which and what part of the model it should be paired with.
# Create dataset object
class BugDatasetBuilder(object):
def __init__(self,
X,
y_head,
y_abdomen,
y_thorax,
batch_size=1,
augmentation_kwargs={'zoom_range':(0.75, 1.25),
'horizontal_flip': True,
'vertical_flip': True,
'rotation_range': 180}):
self.X = X.astype('float32')
self.y_head = y_head.astype('float32')
self.y_abdomen = y_abdomen.astype('float32')
self.y_thorax = y_thorax.astype('float32')
self.batch_size = batch_size
self.augmentation_kwargs = augmentation_kwargs
# Create dataset
self._create_dataset()
def _transform_matrix_offset_center(self, matrix, x, y):
o_x = float(x) / 2 + 0.5
o_y = float(y) / 2 + 0.5
offset_matrix = np.array([[1, 0, o_x], [0, 1, o_y], [0, 0, 1]], dtype='float32')
reset_matrix = np.array([[1, 0, -o_x], [0, 1, -o_y], [0, 0, 1]], dtype='float32')
offset_matrix = tf.convert_to_tensor(offset_matrix)
reset_matrix = tf.convert_to_tensor(reset_matrix)
transform_matrix = tf.keras.backend.dot(tf.keras.backend.dot(offset_matrix, matrix), reset_matrix)
return transform_matrix
def _compute_random_transform_matrix(self):
rotation_range = self.augmentation_kwargs['rotation_range']
zoom_range = self.augmentation_kwargs['zoom_range']
horizontal_flip = self.augmentation_kwargs['horizontal_flip']
vertical_flip = self.augmentation_kwargs['vertical_flip']
# Get random angles
theta = tf.random.uniform(shape=(1,),
minval=-np.pi*rotation_range/180,
maxval=np.pi*rotation_range/180)
one = tf.constant(1.0, shape=(1,))
zero = tf.constant(0.0, shape=(1,))
cos_theta = tf.math.cos(theta)
sin_theta = tf.math.sin(theta)
rot_row_0 = tf.stack([cos_theta, -sin_theta, zero], axis=1)
rot_row_1 = tf.stack([sin_theta, cos_theta, zero], axis=1)
rot_row_2 = tf.stack([zero, zero, one], axis=1)
rotation_matrix = tf.concat([rot_row_0, rot_row_1, rot_row_2], axis=0)
transform_matrix = rotation_matrix
# Get random lr flips
lr = 2*tf.cast(tf.random.categorical(tf.math.log([[0.5, 0.5]]), 1), 'float32')[0] - 1.0
lr_row_0 = tf.stack([lr, zero, zero], axis=1)
lr_row_1 = tf.stack([zero, one, zero], axis=1)
lr_row_2 = tf.stack([zero, zero, one], axis=1)
lr_flip_matrix = tf.concat([lr_row_0, lr_row_1, lr_row_2], axis=0)
transform_matrix = tf.keras.backend.dot(transform_matrix, lr_flip_matrix)
# Get randum ud flips
ud = 2*tf.cast(tf.random.categorical(tf.math.log([[0.5, 0.5]]), 1), 'float32')[0] - 1.0
ud_row_0 = tf.stack([one, zero, zero], axis=1)
ud_row_1 = tf.stack([zero, ud, zero], axis=1)
ud_row_2 = tf.stack([zero, zero, one], axis=1)
ud_flip_matrix = tf.concat([ud_row_0, ud_row_1, ud_row_2], axis=0)
transform_matrix = tf.keras.backend.dot(transform_matrix, ud_flip_matrix)
# Get random zooms
zx = tf.random.uniform(shape=(1,), minval=zoom_range[0], maxval=zoom_range[1])
zy = tf.random.uniform(shape=(1,), minval=zoom_range[0], maxval=zoom_range[1])
z_row_0 = tf.stack([zx, zero, zero], axis=1)
z_row_1 = tf.stack([zero, zy, zero], axis=1)
z_row_2 = tf.stack([zero, zero, one], axis=1)
zoom_matrix = tf.concat([z_row_0, z_row_1, z_row_2], axis=0)
transform_matrix = tf.keras.backend.dot(transform_matrix, zoom_matrix)
# Combine all matrices
h, w = self.X.shape[1], self.X.shape[2]
transform_matrix = self._transform_matrix_offset_center(transform_matrix, h, w)
return transform_matrix
def _augment(self, *args):
X_dict = args[0]
y_dict = args[1]
# Compute random transform matrix
transform_matrix = self._compute_random_transform_matrix()
transform_matrix = tf.reshape(transform_matrix, [1,-1])
transform_matrix = transform_matrix[:,0:8]
for key in X_dict:
X_dict[key] = tfa.image.transform(X_dict[key],
transform_matrix,
interpolation = 'BILINEAR')
for key in y_dict:
interp = 'BILINEAR' if y_dict[key].shape[-1] == 1 else 'NEAREST'
y_dict[key] = tfa.image.transform(y_dict[key],
transform_matrix,
interpolation = interp)
return (X_dict, y_dict)
def _create_dataset(self):
X_train, X_temp, y_head_train, y_head_temp, y_abdomen_train, y_abdomen_temp, y_thorax_train, y_thorax_temp = sklearn.model_selection.train_test_split(self.X, self.y_head, self.y_abdomen, self.y_thorax, train_size=0.8)
X_val, X_test, y_head_val, y_head_test, y_abdomen_val, y_abdomen_test, y_thorax_val, y_thorax_test = sklearn.model_selection.train_test_split(X_temp, y_head_temp, y_abdomen_temp, y_thorax_temp, train_size=0.5)
X_train_dict = {'X': X_train}
y_train_dict = {'head': y_head_train,
'abdomen': y_abdomen_train,
'thorax': y_thorax_train}
X_val_dict = {'X': X_val}
y_val_dict = {'head': y_head_val,
'abdomen': y_abdomen_val,
'thorax': y_thorax_val}
X_test_dict = {'X': X_test}
y_test_dict = {'head': y_head_test,
'abdomen': y_abdomen_test,
'thorax': y_thorax_test}
train_dataset = tf.data.Dataset.from_tensor_slices((X_train_dict, y_train_dict))
val_dataset = tf.data.Dataset.from_tensor_slices((X_val_dict, y_val_dict))
test_dataset = tf.data.Dataset.from_tensor_slices((X_test_dict, y_test_dict))
self.train_dataset = train_dataset.shuffle(256).batch(self.batch_size).map(self._augment)
self.val_dataset = val_dataset.batch(self.batch_size)
self.test_dataset = test_dataset.batch(self.batch_size)
batch_size = 8
bug_data = BugDatasetBuilder(bug_imgs, head_distance, abdomen_distance, thorax_distance, batch_size=batch_size)
fig, axes = plt.subplots(1, 4, figsize=(20,20))
for i in range(4):
X_dict, y_dict = next(iter(bug_data.train_dataset))
axes[0].imshow(X_dict['X'][0,...], cmap='gray')
axes[1].imshow(y_dict['head'][0,...], cmap='jet')
axes[2].imshow(y_dict['abdomen'][0,...], cmap='jet')
axes[3].imshow(y_dict['thorax'][0,...], cmap='jet')
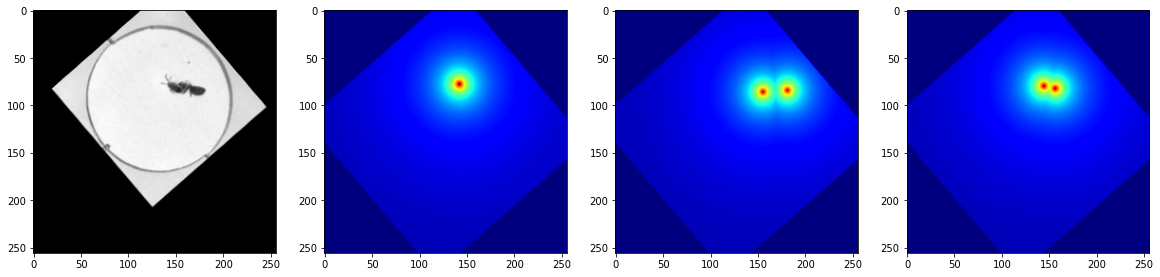
Prepare model#
Next, we will need to make a model. Because we are doing image level prediction, our model choice also becomes a little more complicated. Our backbones will produce features at different scales, and we would like to use them to make dense, pixel level predictions. This requires us to both upsample feature maps and also integrate features across length scales. Lower level features contain fine spatial details while the higher level features contain contextual information - we would like to use both to make our prediction. While there are many approaches to doing so, the two most common are
U-Nets: U-Nets upsample and concatenate to merge feature maps
Feature pyramids: Feature pyramids upsample and add to merge feature maps The accuracy for each approaches are often similar, but feature pyramids are often faster and require less memory.
The first step will be to extract the features from the backbone, which concretely means we need to extract the outputs of specific layers. See the get_backbone function from the deepcell-tf repository for a more general implementation.
def get_backbone(backbone, input_tensor=None, input_shape=None,
use_imagenet=False, return_dict=True,
frames_per_batch=1, **kwargs):
# Make sure backbone name is lower case
_backbone = str(backbone).lower()
# List of acceptable backbones
resnet_backbones = {
'resnet50': tf.keras.applications.resnet.ResNet50,
'resnet101': tf.keras.applications.resnet.ResNet101,
'resnet152': tf.keras.applications.resnet.ResNet152,
}
# Create the input for the model
if input_tensor is not None:
img_input = input_tensor
else:
if input_shape:
img_input = Input(shape=input_shape)
else:
img_input = Input(shape=(None, None, 3))
# Grab the weights if we're using a model pre-trained
# on imagenet
if use_imagenet:
kwargs_with_weights = copy.copy(kwargs)
kwargs_with_weights['weights'] = 'imagenet'
else:
kwargs['weights'] = None
if _backbone in resnet_backbones:
model_cls = resnet_backbones[_backbone]
model = model_cls(input_tensor=img_input, **kwargs)
# Set the weights of the model if requested
if use_imagenet:
model_with_weights = model_cls(**kwargs_with_weights)
model_with_weights.save_weights('model_weights.h5')
model.load_weights('model_weights.h5', by_name=True)
# Define the names of the layers that have the desired features
if _backbone == 'resnet50':
layer_names = ['conv1_relu', 'conv2_block3_out', 'conv3_block4_out',
'conv4_block6_out', 'conv5_block3_out']
elif _backbone == 'resnet101':
layer_names = ['conv1_relu', 'conv2_block3_out', 'conv3_block4_out',
'conv4_block23_out', 'conv5_block3_out']
elif _backbone == 'resnet152':
layer_names = ['conv1_relu', 'conv2_block3_out', 'conv3_block8_out',
'conv4_block36_out', 'conv5_block3_out']
# Get layer outputs
layer_outputs = [model.get_layer(name=ln).output for ln in layer_names]
else:
raise ValueError('Invalid value for `backbone`')
output_dict = {'C{}'.format(i + 1): j for i, j in enumerate(layer_outputs)}
return (model, output_dict) if return_dict else model
With the ability to grab the features from the backbone, the next step is to merge features. Here, we will use a feature pyramid network.
The recipe for how to merge a coarse and fine feature map is shown in the above figure. The coarse feature map is upsampled, while a 1x1 convolution is applied to the fine feature map. The result of each of these operations are then added together. This result is used as the “coarse” feature map for the next step of the pyramid. An implementation of functions to build feature pyramids starting from backbone features is the create_pyramid_features function in deepcell_tf.
Backbone features are typically described using the nomenclature C[n], where n denotes the backbone level. Level n denotes downsampling by $\frac{1}{2^n}$. For example, C3 backbone feature maps would have 1/8th the size of the input image. Feature pyramids typically use features from C3, C4, and C5. The pyramid features of the corresponding level are described as P[n]. The pyramid features derived from C3-C5 are typically P3-P7. Pyramid levels P6 and P7 are created with convolutions of stride 2 of the coarsest backbone feature map.
Note that the typical feature pyramid doesn’t produce feature maps that are the same size of the original image (P3 is 1/8th the size of the input image) - this means we can’t use them to make our pixel-level predictions. To produce correctly sized feature maps, we can upsample the top feature of the feature pyramid using a sequence of upsampling and convolution layers. We could also just add more pyramid levels (e.g. P2, P1, and P0), but this would be computationally more expensive. This sequence of upsampling and convolutions can be viewed as a separate submodel called a head. We can attach three of these heads to our feature pyramid - one for each prediction we hope to make (head, abdomen, and thorax).
from deepcell.layers import ImageNormalization2D, Location2D
from deepcell.model_zoo.fpn import __create_pyramid_features
from tensorflow.keras import backend as K
from tensorflow.keras.layers import Input, Concatenate, Conv2D, Dense
from tensorflow.keras.layers import BatchNormalization, Activation
from tensorflow.keras import Model
from deepcell.utils.misc_utils import get_sorted_keys
from deepcell.model_zoo.fpn import semantic_upsample
def __create_semantic_head(pyramid_dict,
input_target=None,
n_classes=3,
n_filters=128,
n_dense=128,
semantic_id=0,
output_name='prediction_head',
include_top=False,
target_level=2,
upsample_type='upsampling2d',
interpolation='bilinear',
**kwargs):
"""Creates a semantic head from a feature pyramid network.
Args:
pyramid_dict (dict): Dictionary of pyramid names and features.
input_target (tensor): Optional tensor with the input image.
n_classes (int): The number of classes to be predicted.
n_filters (int): The number of convolutional filters.
n_dense (int): Number of dense filters.
semantic_id (int): ID of the semantic head.
ndim (int): The spatial dimensions of the input data.
Must be either 2 or 3.
include_top (bool): Whether to include the final layer of the model
target_level (int): The level we need to reach. Performs
2x upsampling until we're at the target level.
upsample_type (str): Choice of upsampling layer to use from
``['upsamplelike', 'upsampling2d', 'upsampling3d']``.
interpolation (str): Choice of interpolation mode for upsampling
layers from ``['bilinear', 'nearest']``.
Raises:
ValueError: ``interpolation`` not in ``['bilinear', 'nearest']``
ValueError: ``upsample_type`` not in
``['upsamplelike','upsampling2d', 'upsampling3d']``
Returns:
tensorflow.keras.Layer: The semantic segmentation head
"""
# Check input to interpolation
acceptable_interpolation = {'bilinear', 'nearest'}
if interpolation not in acceptable_interpolation:
raise ValueError('Interpolation mode "{}" not supported. '
'Choose from {}.'.format(
interpolation, list(acceptable_interpolation)))
# Check input to upsample_type
acceptable_upsample = {'upsamplelike', 'upsampling2d', 'upsampling3d'}
if upsample_type not in acceptable_upsample:
raise ValueError('Upsample method "{}" not supported. '
'Choose from {}.'.format(
upsample_type, list(acceptable_upsample)))
# Check that there is an input_target if upsamplelike is used
if upsample_type == 'upsamplelike' and input_target is None:
raise ValueError('upsamplelike requires an input_target.')
conv = Conv2D
conv_kernel = (1,1)
channel_axis = -1
if n_classes == 1:
include_top = False
# Get pyramid names and features into list form
pyramid_names = get_sorted_keys(pyramid_dict)
pyramid_features = [pyramid_dict[name] for name in pyramid_names]
# Reverse pyramid names and features
pyramid_names.reverse()
pyramid_features.reverse()
x = pyramid_features[-1]
# Perform upsampling
n_upsample = target_level
x = semantic_upsample(x, n_upsample,
target=input_target, ndim=2,
upsample_type=upsample_type, semantic_id=semantic_id,
interpolation=interpolation)
x = Conv2D(n_dense, conv_kernel, strides=1, padding='same',
name='conv_0_semantic_{}'.format(semantic_id))(x)
x = BatchNormalization(axis=channel_axis,
name='batch_normalization_0_semantic_{}'.format(semantic_id))(x)
x = Activation('relu', name='relu_0_semantic_{}'.format(semantic_id))(x)
# Apply conv and softmax layer
x = Conv2D(n_classes, conv_kernel, strides=1, padding='same',
name='conv_1_semantic_{}'.format(semantic_id))(x)
if include_top:
x = Softmax(axis=channel_axis,
dtype=K.floatx(),
name=output_name)(x)
else:
x = Activation('relu',
dtype=K.floatx(),
name=output_name)(x)
return x
def BugModel(backbone='ResNet50',
input_shape=(256,256,1),
inputs=None,
backbone_levels=['C3', 'C4', 'C5'],
pyramid_levels=['P3', 'P4', 'P5', 'P6', 'P7'],
create_pyramid_features=__create_pyramid_features,
create_semantic_head=__create_semantic_head,
required_channels=3,
norm_method=None,
pooling=None,
location=True,
use_imagenet=True,
lite=False,
upsample_type='upsampling2d',
interpolation='bilinear',
name='bug_model',
**kwargs):
if inputs is None:
inputs = Input(shape=input_shape, name='X')
# Normalize input images
if norm_method is None:
norm = inputs
else:
norm = ImageNormalization2D(norm_method=norm_method,
name='norm')(inputs)
# Add location layer - this breaks translational equivariance
# but provides a notion of location to the model that can help
# improve performance
if location:
loc = Location2D(name='location')(norm)
concat = Concatenate(axis=-1,
name='concat_location')([norm, loc])
else:
concat = norm
# Force the channel size for the backbone input to be 'required_channels'
fixed_inputs = Conv2D(required_channels, (1,1), strides=1,
padding='same', name='conv_channels')(concat)
# Force the input shape
axis = -1
fixed_input_shape = list(input_shape)
fixed_input_shape[axis] = required_channels
fixed_input_shape = tuple(fixed_input_shape)
model_kwargs = {
'include_top': False,
'weights': None,
'input_shape': fixed_input_shape,
'pooling': pooling
}
# Get the backbone features
_, backbone_dict = get_backbone(backbone, fixed_inputs,
use_imagenet=use_imagenet,
return_dict=True,
**model_kwargs)
backbone_dict_reduced = {k: backbone_dict[k] for k in backbone_dict
if k in backbone_levels}
ndim = 2
# Create the feature pyramid and get the relevant features
pyramid_dict = create_pyramid_features(backbone_dict_reduced,
ndim=ndim,
lite=lite,
interpolation=interpolation,
upsample_type=upsample_type,
z_axis_convolutions=False)
features = [pyramid_dict[key] for key in pyramid_levels]
# Figure out how much upsampling is required (e.g., if the top layer
# is P3, then a 8X upsample is required)
semantic_levels = [int(re.findall(r'\d+', k)[0]) for k in pyramid_dict]
target_level = min(semantic_levels)
# Create the heads that perform upsampling to perform the final prediction
prediction_head_list = []
head_head = create_semantic_head(pyramid_dict, n_classes=1,
input_target=inputs, target_level=target_level, semantic_id=0,
output_name='head', ndim=ndim, upsample_type=upsample_type,
interpolation=interpolation, **kwargs)
abdomen_head = create_semantic_head(pyramid_dict, n_classes=1,
input_target=inputs, target_level=target_level, semantic_id=1,
output_name='abdomen', ndim=ndim, upsample_type=upsample_type,
interpolation=interpolation, **kwargs)
thorax_head = create_semantic_head(pyramid_dict, n_classes=1,
input_target=inputs, target_level=target_level, semantic_id=2,
output_name='thorax', ndim=ndim, upsample_type=upsample_type,
interpolation=interpolation, **kwargs)
outputs = [head_head, abdomen_head, thorax_head]
model = Model(inputs=inputs, outputs=outputs, name=name)
return model
bug_model = BugModel()
bug_model.summary()
Show code cell output
Model: "bug_model"
__________________________________________________________________________________________________
Layer (type) Output Shape Param # Connected to
==================================================================================================
X (InputLayer) [(None, 256, 256, 1) 0
__________________________________________________________________________________________________
location (Location2D) (None, None, None, 2 0 X[0][0]
__________________________________________________________________________________________________
concat_location (Concatenate) (None, 256, 256, 3) 0 X[0][0]
location[0][0]
__________________________________________________________________________________________________
conv_channels (Conv2D) (None, 256, 256, 3) 12 concat_location[0][0]
__________________________________________________________________________________________________
conv1_pad (ZeroPadding2D) (None, 262, 262, 3) 0 conv_channels[0][0]
__________________________________________________________________________________________________
conv1_conv (Conv2D) (None, 128, 128, 64) 9472 conv1_pad[0][0]
__________________________________________________________________________________________________
conv1_bn (BatchNormalization) (None, 128, 128, 64) 256 conv1_conv[0][0]
__________________________________________________________________________________________________
conv1_relu (Activation) (None, 128, 128, 64) 0 conv1_bn[0][0]
__________________________________________________________________________________________________
pool1_pad (ZeroPadding2D) (None, 130, 130, 64) 0 conv1_relu[0][0]
__________________________________________________________________________________________________
pool1_pool (MaxPooling2D) (None, 64, 64, 64) 0 pool1_pad[0][0]
__________________________________________________________________________________________________
conv2_block1_1_conv (Conv2D) (None, 64, 64, 64) 4160 pool1_pool[0][0]
__________________________________________________________________________________________________
conv2_block1_1_bn (BatchNormali (None, 64, 64, 64) 256 conv2_block1_1_conv[0][0]
__________________________________________________________________________________________________
conv2_block1_1_relu (Activation (None, 64, 64, 64) 0 conv2_block1_1_bn[0][0]
__________________________________________________________________________________________________
conv2_block1_2_conv (Conv2D) (None, 64, 64, 64) 36928 conv2_block1_1_relu[0][0]
__________________________________________________________________________________________________
conv2_block1_2_bn (BatchNormali (None, 64, 64, 64) 256 conv2_block1_2_conv[0][0]
__________________________________________________________________________________________________
conv2_block1_2_relu (Activation (None, 64, 64, 64) 0 conv2_block1_2_bn[0][0]
__________________________________________________________________________________________________
conv2_block1_0_conv (Conv2D) (None, 64, 64, 256) 16640 pool1_pool[0][0]
__________________________________________________________________________________________________
conv2_block1_3_conv (Conv2D) (None, 64, 64, 256) 16640 conv2_block1_2_relu[0][0]
__________________________________________________________________________________________________
conv2_block1_0_bn (BatchNormali (None, 64, 64, 256) 1024 conv2_block1_0_conv[0][0]
__________________________________________________________________________________________________
conv2_block1_3_bn (BatchNormali (None, 64, 64, 256) 1024 conv2_block1_3_conv[0][0]
__________________________________________________________________________________________________
conv2_block1_add (Add) (None, 64, 64, 256) 0 conv2_block1_0_bn[0][0]
conv2_block1_3_bn[0][0]
__________________________________________________________________________________________________
conv2_block1_out (Activation) (None, 64, 64, 256) 0 conv2_block1_add[0][0]
__________________________________________________________________________________________________
conv2_block2_1_conv (Conv2D) (None, 64, 64, 64) 16448 conv2_block1_out[0][0]
__________________________________________________________________________________________________
conv2_block2_1_bn (BatchNormali (None, 64, 64, 64) 256 conv2_block2_1_conv[0][0]
__________________________________________________________________________________________________
conv2_block2_1_relu (Activation (None, 64, 64, 64) 0 conv2_block2_1_bn[0][0]
__________________________________________________________________________________________________
conv2_block2_2_conv (Conv2D) (None, 64, 64, 64) 36928 conv2_block2_1_relu[0][0]
__________________________________________________________________________________________________
conv2_block2_2_bn (BatchNormali (None, 64, 64, 64) 256 conv2_block2_2_conv[0][0]
__________________________________________________________________________________________________
conv2_block2_2_relu (Activation (None, 64, 64, 64) 0 conv2_block2_2_bn[0][0]
__________________________________________________________________________________________________
conv2_block2_3_conv (Conv2D) (None, 64, 64, 256) 16640 conv2_block2_2_relu[0][0]
__________________________________________________________________________________________________
conv2_block2_3_bn (BatchNormali (None, 64, 64, 256) 1024 conv2_block2_3_conv[0][0]
__________________________________________________________________________________________________
conv2_block2_add (Add) (None, 64, 64, 256) 0 conv2_block1_out[0][0]
conv2_block2_3_bn[0][0]
__________________________________________________________________________________________________
conv2_block2_out (Activation) (None, 64, 64, 256) 0 conv2_block2_add[0][0]
__________________________________________________________________________________________________
conv2_block3_1_conv (Conv2D) (None, 64, 64, 64) 16448 conv2_block2_out[0][0]
__________________________________________________________________________________________________
conv2_block3_1_bn (BatchNormali (None, 64, 64, 64) 256 conv2_block3_1_conv[0][0]
__________________________________________________________________________________________________
conv2_block3_1_relu (Activation (None, 64, 64, 64) 0 conv2_block3_1_bn[0][0]
__________________________________________________________________________________________________
conv2_block3_2_conv (Conv2D) (None, 64, 64, 64) 36928 conv2_block3_1_relu[0][0]
__________________________________________________________________________________________________
conv2_block3_2_bn (BatchNormali (None, 64, 64, 64) 256 conv2_block3_2_conv[0][0]
__________________________________________________________________________________________________
conv2_block3_2_relu (Activation (None, 64, 64, 64) 0 conv2_block3_2_bn[0][0]
__________________________________________________________________________________________________
conv2_block3_3_conv (Conv2D) (None, 64, 64, 256) 16640 conv2_block3_2_relu[0][0]
__________________________________________________________________________________________________
conv2_block3_3_bn (BatchNormali (None, 64, 64, 256) 1024 conv2_block3_3_conv[0][0]
__________________________________________________________________________________________________
conv2_block3_add (Add) (None, 64, 64, 256) 0 conv2_block2_out[0][0]
conv2_block3_3_bn[0][0]
__________________________________________________________________________________________________
conv2_block3_out (Activation) (None, 64, 64, 256) 0 conv2_block3_add[0][0]
__________________________________________________________________________________________________
conv3_block1_1_conv (Conv2D) (None, 32, 32, 128) 32896 conv2_block3_out[0][0]
__________________________________________________________________________________________________
conv3_block1_1_bn (BatchNormali (None, 32, 32, 128) 512 conv3_block1_1_conv[0][0]
__________________________________________________________________________________________________
conv3_block1_1_relu (Activation (None, 32, 32, 128) 0 conv3_block1_1_bn[0][0]
__________________________________________________________________________________________________
conv3_block1_2_conv (Conv2D) (None, 32, 32, 128) 147584 conv3_block1_1_relu[0][0]
__________________________________________________________________________________________________
conv3_block1_2_bn (BatchNormali (None, 32, 32, 128) 512 conv3_block1_2_conv[0][0]
__________________________________________________________________________________________________
conv3_block1_2_relu (Activation (None, 32, 32, 128) 0 conv3_block1_2_bn[0][0]
__________________________________________________________________________________________________
conv3_block1_0_conv (Conv2D) (None, 32, 32, 512) 131584 conv2_block3_out[0][0]
__________________________________________________________________________________________________
conv3_block1_3_conv (Conv2D) (None, 32, 32, 512) 66048 conv3_block1_2_relu[0][0]
__________________________________________________________________________________________________
conv3_block1_0_bn (BatchNormali (None, 32, 32, 512) 2048 conv3_block1_0_conv[0][0]
__________________________________________________________________________________________________
conv3_block1_3_bn (BatchNormali (None, 32, 32, 512) 2048 conv3_block1_3_conv[0][0]
__________________________________________________________________________________________________
conv3_block1_add (Add) (None, 32, 32, 512) 0 conv3_block1_0_bn[0][0]
conv3_block1_3_bn[0][0]
__________________________________________________________________________________________________
conv3_block1_out (Activation) (None, 32, 32, 512) 0 conv3_block1_add[0][0]
__________________________________________________________________________________________________
conv3_block2_1_conv (Conv2D) (None, 32, 32, 128) 65664 conv3_block1_out[0][0]
__________________________________________________________________________________________________
conv3_block2_1_bn (BatchNormali (None, 32, 32, 128) 512 conv3_block2_1_conv[0][0]
__________________________________________________________________________________________________
conv3_block2_1_relu (Activation (None, 32, 32, 128) 0 conv3_block2_1_bn[0][0]
__________________________________________________________________________________________________
conv3_block2_2_conv (Conv2D) (None, 32, 32, 128) 147584 conv3_block2_1_relu[0][0]
__________________________________________________________________________________________________
conv3_block2_2_bn (BatchNormali (None, 32, 32, 128) 512 conv3_block2_2_conv[0][0]
__________________________________________________________________________________________________
conv3_block2_2_relu (Activation (None, 32, 32, 128) 0 conv3_block2_2_bn[0][0]
__________________________________________________________________________________________________
conv3_block2_3_conv (Conv2D) (None, 32, 32, 512) 66048 conv3_block2_2_relu[0][0]
__________________________________________________________________________________________________
conv3_block2_3_bn (BatchNormali (None, 32, 32, 512) 2048 conv3_block2_3_conv[0][0]
__________________________________________________________________________________________________
conv3_block2_add (Add) (None, 32, 32, 512) 0 conv3_block1_out[0][0]
conv3_block2_3_bn[0][0]
__________________________________________________________________________________________________
conv3_block2_out (Activation) (None, 32, 32, 512) 0 conv3_block2_add[0][0]
__________________________________________________________________________________________________
conv3_block3_1_conv (Conv2D) (None, 32, 32, 128) 65664 conv3_block2_out[0][0]
__________________________________________________________________________________________________
conv3_block3_1_bn (BatchNormali (None, 32, 32, 128) 512 conv3_block3_1_conv[0][0]
__________________________________________________________________________________________________
conv3_block3_1_relu (Activation (None, 32, 32, 128) 0 conv3_block3_1_bn[0][0]
__________________________________________________________________________________________________
conv3_block3_2_conv (Conv2D) (None, 32, 32, 128) 147584 conv3_block3_1_relu[0][0]
__________________________________________________________________________________________________
conv3_block3_2_bn (BatchNormali (None, 32, 32, 128) 512 conv3_block3_2_conv[0][0]
__________________________________________________________________________________________________
conv3_block3_2_relu (Activation (None, 32, 32, 128) 0 conv3_block3_2_bn[0][0]
__________________________________________________________________________________________________
conv3_block3_3_conv (Conv2D) (None, 32, 32, 512) 66048 conv3_block3_2_relu[0][0]
__________________________________________________________________________________________________
conv3_block3_3_bn (BatchNormali (None, 32, 32, 512) 2048 conv3_block3_3_conv[0][0]
__________________________________________________________________________________________________
conv3_block3_add (Add) (None, 32, 32, 512) 0 conv3_block2_out[0][0]
conv3_block3_3_bn[0][0]
__________________________________________________________________________________________________
conv3_block3_out (Activation) (None, 32, 32, 512) 0 conv3_block3_add[0][0]
__________________________________________________________________________________________________
conv3_block4_1_conv (Conv2D) (None, 32, 32, 128) 65664 conv3_block3_out[0][0]
__________________________________________________________________________________________________
conv3_block4_1_bn (BatchNormali (None, 32, 32, 128) 512 conv3_block4_1_conv[0][0]
__________________________________________________________________________________________________
conv3_block4_1_relu (Activation (None, 32, 32, 128) 0 conv3_block4_1_bn[0][0]
__________________________________________________________________________________________________
conv3_block4_2_conv (Conv2D) (None, 32, 32, 128) 147584 conv3_block4_1_relu[0][0]
__________________________________________________________________________________________________
conv3_block4_2_bn (BatchNormali (None, 32, 32, 128) 512 conv3_block4_2_conv[0][0]
__________________________________________________________________________________________________
conv3_block4_2_relu (Activation (None, 32, 32, 128) 0 conv3_block4_2_bn[0][0]
__________________________________________________________________________________________________
conv3_block4_3_conv (Conv2D) (None, 32, 32, 512) 66048 conv3_block4_2_relu[0][0]
__________________________________________________________________________________________________
conv3_block4_3_bn (BatchNormali (None, 32, 32, 512) 2048 conv3_block4_3_conv[0][0]
__________________________________________________________________________________________________
conv3_block4_add (Add) (None, 32, 32, 512) 0 conv3_block3_out[0][0]
conv3_block4_3_bn[0][0]
__________________________________________________________________________________________________
conv3_block4_out (Activation) (None, 32, 32, 512) 0 conv3_block4_add[0][0]
__________________________________________________________________________________________________
conv4_block1_1_conv (Conv2D) (None, 16, 16, 256) 131328 conv3_block4_out[0][0]
__________________________________________________________________________________________________
conv4_block1_1_bn (BatchNormali (None, 16, 16, 256) 1024 conv4_block1_1_conv[0][0]
__________________________________________________________________________________________________
conv4_block1_1_relu (Activation (None, 16, 16, 256) 0 conv4_block1_1_bn[0][0]
__________________________________________________________________________________________________
conv4_block1_2_conv (Conv2D) (None, 16, 16, 256) 590080 conv4_block1_1_relu[0][0]
__________________________________________________________________________________________________
conv4_block1_2_bn (BatchNormali (None, 16, 16, 256) 1024 conv4_block1_2_conv[0][0]
__________________________________________________________________________________________________
conv4_block1_2_relu (Activation (None, 16, 16, 256) 0 conv4_block1_2_bn[0][0]
__________________________________________________________________________________________________
conv4_block1_0_conv (Conv2D) (None, 16, 16, 1024) 525312 conv3_block4_out[0][0]
__________________________________________________________________________________________________
conv4_block1_3_conv (Conv2D) (None, 16, 16, 1024) 263168 conv4_block1_2_relu[0][0]
__________________________________________________________________________________________________
conv4_block1_0_bn (BatchNormali (None, 16, 16, 1024) 4096 conv4_block1_0_conv[0][0]
__________________________________________________________________________________________________
conv4_block1_3_bn (BatchNormali (None, 16, 16, 1024) 4096 conv4_block1_3_conv[0][0]
__________________________________________________________________________________________________
conv4_block1_add (Add) (None, 16, 16, 1024) 0 conv4_block1_0_bn[0][0]
conv4_block1_3_bn[0][0]
__________________________________________________________________________________________________
conv4_block1_out (Activation) (None, 16, 16, 1024) 0 conv4_block1_add[0][0]
__________________________________________________________________________________________________
conv4_block2_1_conv (Conv2D) (None, 16, 16, 256) 262400 conv4_block1_out[0][0]
__________________________________________________________________________________________________
conv4_block2_1_bn (BatchNormali (None, 16, 16, 256) 1024 conv4_block2_1_conv[0][0]
__________________________________________________________________________________________________
conv4_block2_1_relu (Activation (None, 16, 16, 256) 0 conv4_block2_1_bn[0][0]
__________________________________________________________________________________________________
conv4_block2_2_conv (Conv2D) (None, 16, 16, 256) 590080 conv4_block2_1_relu[0][0]
__________________________________________________________________________________________________
conv4_block2_2_bn (BatchNormali (None, 16, 16, 256) 1024 conv4_block2_2_conv[0][0]
__________________________________________________________________________________________________
conv4_block2_2_relu (Activation (None, 16, 16, 256) 0 conv4_block2_2_bn[0][0]
__________________________________________________________________________________________________
conv4_block2_3_conv (Conv2D) (None, 16, 16, 1024) 263168 conv4_block2_2_relu[0][0]
__________________________________________________________________________________________________
conv4_block2_3_bn (BatchNormali (None, 16, 16, 1024) 4096 conv4_block2_3_conv[0][0]
__________________________________________________________________________________________________
conv4_block2_add (Add) (None, 16, 16, 1024) 0 conv4_block1_out[0][0]
conv4_block2_3_bn[0][0]
__________________________________________________________________________________________________
conv4_block2_out (Activation) (None, 16, 16, 1024) 0 conv4_block2_add[0][0]
__________________________________________________________________________________________________
conv4_block3_1_conv (Conv2D) (None, 16, 16, 256) 262400 conv4_block2_out[0][0]
__________________________________________________________________________________________________
conv4_block3_1_bn (BatchNormali (None, 16, 16, 256) 1024 conv4_block3_1_conv[0][0]
__________________________________________________________________________________________________
conv4_block3_1_relu (Activation (None, 16, 16, 256) 0 conv4_block3_1_bn[0][0]
__________________________________________________________________________________________________
conv4_block3_2_conv (Conv2D) (None, 16, 16, 256) 590080 conv4_block3_1_relu[0][0]
__________________________________________________________________________________________________
conv4_block3_2_bn (BatchNormali (None, 16, 16, 256) 1024 conv4_block3_2_conv[0][0]
__________________________________________________________________________________________________
conv4_block3_2_relu (Activation (None, 16, 16, 256) 0 conv4_block3_2_bn[0][0]
__________________________________________________________________________________________________
conv4_block3_3_conv (Conv2D) (None, 16, 16, 1024) 263168 conv4_block3_2_relu[0][0]
__________________________________________________________________________________________________
conv4_block3_3_bn (BatchNormali (None, 16, 16, 1024) 4096 conv4_block3_3_conv[0][0]
__________________________________________________________________________________________________
conv4_block3_add (Add) (None, 16, 16, 1024) 0 conv4_block2_out[0][0]
conv4_block3_3_bn[0][0]
__________________________________________________________________________________________________
conv4_block3_out (Activation) (None, 16, 16, 1024) 0 conv4_block3_add[0][0]
__________________________________________________________________________________________________
conv4_block4_1_conv (Conv2D) (None, 16, 16, 256) 262400 conv4_block3_out[0][0]
__________________________________________________________________________________________________
conv4_block4_1_bn (BatchNormali (None, 16, 16, 256) 1024 conv4_block4_1_conv[0][0]
__________________________________________________________________________________________________
conv4_block4_1_relu (Activation (None, 16, 16, 256) 0 conv4_block4_1_bn[0][0]
__________________________________________________________________________________________________
conv4_block4_2_conv (Conv2D) (None, 16, 16, 256) 590080 conv4_block4_1_relu[0][0]
__________________________________________________________________________________________________
conv4_block4_2_bn (BatchNormali (None, 16, 16, 256) 1024 conv4_block4_2_conv[0][0]
__________________________________________________________________________________________________
conv4_block4_2_relu (Activation (None, 16, 16, 256) 0 conv4_block4_2_bn[0][0]
__________________________________________________________________________________________________
conv4_block4_3_conv (Conv2D) (None, 16, 16, 1024) 263168 conv4_block4_2_relu[0][0]
__________________________________________________________________________________________________
conv4_block4_3_bn (BatchNormali (None, 16, 16, 1024) 4096 conv4_block4_3_conv[0][0]
__________________________________________________________________________________________________
conv4_block4_add (Add) (None, 16, 16, 1024) 0 conv4_block3_out[0][0]
conv4_block4_3_bn[0][0]
__________________________________________________________________________________________________
conv4_block4_out (Activation) (None, 16, 16, 1024) 0 conv4_block4_add[0][0]
__________________________________________________________________________________________________
conv4_block5_1_conv (Conv2D) (None, 16, 16, 256) 262400 conv4_block4_out[0][0]
__________________________________________________________________________________________________
conv4_block5_1_bn (BatchNormali (None, 16, 16, 256) 1024 conv4_block5_1_conv[0][0]
__________________________________________________________________________________________________
conv4_block5_1_relu (Activation (None, 16, 16, 256) 0 conv4_block5_1_bn[0][0]
__________________________________________________________________________________________________
conv4_block5_2_conv (Conv2D) (None, 16, 16, 256) 590080 conv4_block5_1_relu[0][0]
__________________________________________________________________________________________________
conv4_block5_2_bn (BatchNormali (None, 16, 16, 256) 1024 conv4_block5_2_conv[0][0]
__________________________________________________________________________________________________
conv4_block5_2_relu (Activation (None, 16, 16, 256) 0 conv4_block5_2_bn[0][0]
__________________________________________________________________________________________________
conv4_block5_3_conv (Conv2D) (None, 16, 16, 1024) 263168 conv4_block5_2_relu[0][0]
__________________________________________________________________________________________________
conv4_block5_3_bn (BatchNormali (None, 16, 16, 1024) 4096 conv4_block5_3_conv[0][0]
__________________________________________________________________________________________________
conv4_block5_add (Add) (None, 16, 16, 1024) 0 conv4_block4_out[0][0]
conv4_block5_3_bn[0][0]
__________________________________________________________________________________________________
conv4_block5_out (Activation) (None, 16, 16, 1024) 0 conv4_block5_add[0][0]
__________________________________________________________________________________________________
conv4_block6_1_conv (Conv2D) (None, 16, 16, 256) 262400 conv4_block5_out[0][0]
__________________________________________________________________________________________________
conv4_block6_1_bn (BatchNormali (None, 16, 16, 256) 1024 conv4_block6_1_conv[0][0]
__________________________________________________________________________________________________
conv4_block6_1_relu (Activation (None, 16, 16, 256) 0 conv4_block6_1_bn[0][0]
__________________________________________________________________________________________________
conv4_block6_2_conv (Conv2D) (None, 16, 16, 256) 590080 conv4_block6_1_relu[0][0]
__________________________________________________________________________________________________
conv4_block6_2_bn (BatchNormali (None, 16, 16, 256) 1024 conv4_block6_2_conv[0][0]
__________________________________________________________________________________________________
conv4_block6_2_relu (Activation (None, 16, 16, 256) 0 conv4_block6_2_bn[0][0]
__________________________________________________________________________________________________
conv4_block6_3_conv (Conv2D) (None, 16, 16, 1024) 263168 conv4_block6_2_relu[0][0]
__________________________________________________________________________________________________
conv4_block6_3_bn (BatchNormali (None, 16, 16, 1024) 4096 conv4_block6_3_conv[0][0]
__________________________________________________________________________________________________
conv4_block6_add (Add) (None, 16, 16, 1024) 0 conv4_block5_out[0][0]
conv4_block6_3_bn[0][0]
__________________________________________________________________________________________________
conv4_block6_out (Activation) (None, 16, 16, 1024) 0 conv4_block6_add[0][0]
__________________________________________________________________________________________________
conv5_block1_1_conv (Conv2D) (None, 8, 8, 512) 524800 conv4_block6_out[0][0]
__________________________________________________________________________________________________
conv5_block1_1_bn (BatchNormali (None, 8, 8, 512) 2048 conv5_block1_1_conv[0][0]
__________________________________________________________________________________________________
conv5_block1_1_relu (Activation (None, 8, 8, 512) 0 conv5_block1_1_bn[0][0]
__________________________________________________________________________________________________
conv5_block1_2_conv (Conv2D) (None, 8, 8, 512) 2359808 conv5_block1_1_relu[0][0]
__________________________________________________________________________________________________
conv5_block1_2_bn (BatchNormali (None, 8, 8, 512) 2048 conv5_block1_2_conv[0][0]
__________________________________________________________________________________________________
conv5_block1_2_relu (Activation (None, 8, 8, 512) 0 conv5_block1_2_bn[0][0]
__________________________________________________________________________________________________
conv5_block1_0_conv (Conv2D) (None, 8, 8, 2048) 2099200 conv4_block6_out[0][0]
__________________________________________________________________________________________________
conv5_block1_3_conv (Conv2D) (None, 8, 8, 2048) 1050624 conv5_block1_2_relu[0][0]
__________________________________________________________________________________________________
conv5_block1_0_bn (BatchNormali (None, 8, 8, 2048) 8192 conv5_block1_0_conv[0][0]
__________________________________________________________________________________________________
conv5_block1_3_bn (BatchNormali (None, 8, 8, 2048) 8192 conv5_block1_3_conv[0][0]
__________________________________________________________________________________________________
conv5_block1_add (Add) (None, 8, 8, 2048) 0 conv5_block1_0_bn[0][0]
conv5_block1_3_bn[0][0]
__________________________________________________________________________________________________
conv5_block1_out (Activation) (None, 8, 8, 2048) 0 conv5_block1_add[0][0]
__________________________________________________________________________________________________
conv5_block2_1_conv (Conv2D) (None, 8, 8, 512) 1049088 conv5_block1_out[0][0]
__________________________________________________________________________________________________
conv5_block2_1_bn (BatchNormali (None, 8, 8, 512) 2048 conv5_block2_1_conv[0][0]
__________________________________________________________________________________________________
conv5_block2_1_relu (Activation (None, 8, 8, 512) 0 conv5_block2_1_bn[0][0]
__________________________________________________________________________________________________
conv5_block2_2_conv (Conv2D) (None, 8, 8, 512) 2359808 conv5_block2_1_relu[0][0]
__________________________________________________________________________________________________
conv5_block2_2_bn (BatchNormali (None, 8, 8, 512) 2048 conv5_block2_2_conv[0][0]
__________________________________________________________________________________________________
conv5_block2_2_relu (Activation (None, 8, 8, 512) 0 conv5_block2_2_bn[0][0]
__________________________________________________________________________________________________
conv5_block2_3_conv (Conv2D) (None, 8, 8, 2048) 1050624 conv5_block2_2_relu[0][0]
__________________________________________________________________________________________________
conv5_block2_3_bn (BatchNormali (None, 8, 8, 2048) 8192 conv5_block2_3_conv[0][0]
__________________________________________________________________________________________________
conv5_block2_add (Add) (None, 8, 8, 2048) 0 conv5_block1_out[0][0]
conv5_block2_3_bn[0][0]
__________________________________________________________________________________________________
conv5_block2_out (Activation) (None, 8, 8, 2048) 0 conv5_block2_add[0][0]
__________________________________________________________________________________________________
conv5_block3_1_conv (Conv2D) (None, 8, 8, 512) 1049088 conv5_block2_out[0][0]
__________________________________________________________________________________________________
conv5_block3_1_bn (BatchNormali (None, 8, 8, 512) 2048 conv5_block3_1_conv[0][0]
__________________________________________________________________________________________________
conv5_block3_1_relu (Activation (None, 8, 8, 512) 0 conv5_block3_1_bn[0][0]
__________________________________________________________________________________________________
conv5_block3_2_conv (Conv2D) (None, 8, 8, 512) 2359808 conv5_block3_1_relu[0][0]
__________________________________________________________________________________________________
conv5_block3_2_bn (BatchNormali (None, 8, 8, 512) 2048 conv5_block3_2_conv[0][0]
__________________________________________________________________________________________________
conv5_block3_2_relu (Activation (None, 8, 8, 512) 0 conv5_block3_2_bn[0][0]
__________________________________________________________________________________________________
conv5_block3_3_conv (Conv2D) (None, 8, 8, 2048) 1050624 conv5_block3_2_relu[0][0]
__________________________________________________________________________________________________
conv5_block3_3_bn (BatchNormali (None, 8, 8, 2048) 8192 conv5_block3_3_conv[0][0]
__________________________________________________________________________________________________
conv5_block3_add (Add) (None, 8, 8, 2048) 0 conv5_block2_out[0][0]
conv5_block3_3_bn[0][0]
__________________________________________________________________________________________________
conv5_block3_out (Activation) (None, 8, 8, 2048) 0 conv5_block3_add[0][0]
__________________________________________________________________________________________________
C5_reduced (Conv2D) (None, 8, 8, 256) 524544 conv5_block3_out[0][0]
__________________________________________________________________________________________________
C4_reduced (Conv2D) (None, 16, 16, 256) 262400 conv4_block6_out[0][0]
__________________________________________________________________________________________________
P5_upsampled (UpSampling2D) (None, 16, 16, 256) 0 C5_reduced[0][0]
__________________________________________________________________________________________________
P4_merged (Add) (None, 16, 16, 256) 0 C4_reduced[0][0]
P5_upsampled[0][0]
__________________________________________________________________________________________________
C3_reduced (Conv2D) (None, 32, 32, 256) 131328 conv3_block4_out[0][0]
__________________________________________________________________________________________________
P4_upsampled (UpSampling2D) (None, 32, 32, 256) 0 P4_merged[0][0]
__________________________________________________________________________________________________
P3_merged (Add) (None, 32, 32, 256) 0 C3_reduced[0][0]
P4_upsampled[0][0]
__________________________________________________________________________________________________
P3 (Conv2D) (None, 32, 32, 256) 590080 P3_merged[0][0]
__________________________________________________________________________________________________
conv_0_semantic_upsample_0 (Con (None, 32, 32, 64) 147520 P3[0][0]
__________________________________________________________________________________________________
conv_0_semantic_upsample_1 (Con (None, 32, 32, 64) 147520 P3[0][0]
__________________________________________________________________________________________________
conv_0_semantic_upsample_2 (Con (None, 32, 32, 64) 147520 P3[0][0]
__________________________________________________________________________________________________
upsampling_0_semantic_upsample_ (None, 64, 64, 64) 0 conv_0_semantic_upsample_0[0][0]
__________________________________________________________________________________________________
upsampling_0_semantic_upsample_ (None, 64, 64, 64) 0 conv_0_semantic_upsample_1[0][0]
__________________________________________________________________________________________________
upsampling_0_semantic_upsample_ (None, 64, 64, 64) 0 conv_0_semantic_upsample_2[0][0]
__________________________________________________________________________________________________
conv_1_semantic_upsample_0 (Con (None, 64, 64, 64) 36928 upsampling_0_semantic_upsample_0[
__________________________________________________________________________________________________
conv_1_semantic_upsample_1 (Con (None, 64, 64, 64) 36928 upsampling_0_semantic_upsample_1[
__________________________________________________________________________________________________
conv_1_semantic_upsample_2 (Con (None, 64, 64, 64) 36928 upsampling_0_semantic_upsample_2[
__________________________________________________________________________________________________
upsampling_1_semantic_upsample_ (None, 128, 128, 64) 0 conv_1_semantic_upsample_0[0][0]
__________________________________________________________________________________________________
upsampling_1_semantic_upsample_ (None, 128, 128, 64) 0 conv_1_semantic_upsample_1[0][0]
__________________________________________________________________________________________________
upsampling_1_semantic_upsample_ (None, 128, 128, 64) 0 conv_1_semantic_upsample_2[0][0]
__________________________________________________________________________________________________
conv_2_semantic_upsample_0 (Con (None, 128, 128, 64) 36928 upsampling_1_semantic_upsample_0[
__________________________________________________________________________________________________
conv_2_semantic_upsample_1 (Con (None, 128, 128, 64) 36928 upsampling_1_semantic_upsample_1[
__________________________________________________________________________________________________
conv_2_semantic_upsample_2 (Con (None, 128, 128, 64) 36928 upsampling_1_semantic_upsample_2[
__________________________________________________________________________________________________
upsampling_2_semantic_upsample_ (None, 256, 256, 64) 0 conv_2_semantic_upsample_0[0][0]
__________________________________________________________________________________________________
upsampling_2_semantic_upsample_ (None, 256, 256, 64) 0 conv_2_semantic_upsample_1[0][0]
__________________________________________________________________________________________________
upsampling_2_semantic_upsample_ (None, 256, 256, 64) 0 conv_2_semantic_upsample_2[0][0]
__________________________________________________________________________________________________
conv_0_semantic_0 (Conv2D) (None, 256, 256, 128 8320 upsampling_2_semantic_upsample_0[
__________________________________________________________________________________________________
conv_0_semantic_1 (Conv2D) (None, 256, 256, 128 8320 upsampling_2_semantic_upsample_1[
__________________________________________________________________________________________________
conv_0_semantic_2 (Conv2D) (None, 256, 256, 128 8320 upsampling_2_semantic_upsample_2[
__________________________________________________________________________________________________
batch_normalization_0_semantic_ (None, 256, 256, 128 512 conv_0_semantic_0[0][0]
__________________________________________________________________________________________________
batch_normalization_0_semantic_ (None, 256, 256, 128 512 conv_0_semantic_1[0][0]
__________________________________________________________________________________________________
batch_normalization_0_semantic_ (None, 256, 256, 128 512 conv_0_semantic_2[0][0]
__________________________________________________________________________________________________
relu_0_semantic_0 (Activation) (None, 256, 256, 128 0 batch_normalization_0_semantic_0[
__________________________________________________________________________________________________
relu_0_semantic_1 (Activation) (None, 256, 256, 128 0 batch_normalization_0_semantic_1[
__________________________________________________________________________________________________
relu_0_semantic_2 (Activation) (None, 256, 256, 128 0 batch_normalization_0_semantic_2[
__________________________________________________________________________________________________
conv_1_semantic_0 (Conv2D) (None, 256, 256, 1) 129 relu_0_semantic_0[0][0]
__________________________________________________________________________________________________
conv_1_semantic_1 (Conv2D) (None, 256, 256, 1) 129 relu_0_semantic_1[0][0]
__________________________________________________________________________________________________
conv_1_semantic_2 (Conv2D) (None, 256, 256, 1) 129 relu_0_semantic_2[0][0]
__________________________________________________________________________________________________
head (Activation) (None, 256, 256, 1) 0 conv_1_semantic_0[0][0]
__________________________________________________________________________________________________
abdomen (Activation) (None, 256, 256, 1) 0 conv_1_semantic_1[0][0]
__________________________________________________________________________________________________
thorax (Activation) (None, 256, 256, 1) 0 conv_1_semantic_2[0][0]
==================================================================================================
Total params: 25,787,087
Trainable params: 25,733,199
Non-trainable params: 53,888
__________________________________________________________________________________________________
Train Model#
from tensorflow.keras.losses import MSE
from tensorflow.keras.optimizers import SGD, Adam
# Define loss functions
loss = {}
for layer in bug_model.layers:
if layer.name in ['head', 'abdomen', 'thorax']:
loss[layer.name] = MSE
# Define training parameters
n_epochs = 16
lr = 1e-4
optimizer = Adam(lr=lr, clipnorm=0.001)
# Compile model
bug_model.compile(loss=loss, optimizer=optimizer)
# Define callbacks
bug_model_path = '/notebooks/bebi205-sandbox/beetles/spot-detection-beetles.h5'
bug_callbacks = [tf.keras.callbacks.ModelCheckpoint(
bug_model_path, monitor='val_loss',
save_best_only=True, verbose=1,
save_weights_only=True)
]
bug_callbacks.append(tf.keras.callbacks.ReduceLROnPlateau(
monitor='val_loss', factor=0.5, verbose=1,
patience=3, min_lr=1e-7)
)
# Train model
loss_history = bug_model.fit(bug_data.train_dataset,
validation_data=bug_data.val_dataset,
epochs=n_epochs,
verbose=1,
callbacks=bug_callbacks)
Epoch 1/16
85/85 [==============================] - 41s 293ms/step - loss: 0.1795 - head_loss: 0.0184 - abdomen_loss: 0.1233 - thorax_loss: 0.0378 - val_loss: 0.0633 - val_head_loss: 0.0210 - val_abdomen_loss: 0.0214 - val_thorax_loss: 0.0209
Epoch 00001: val_loss improved from inf to 0.06329, saving model to /notebooks/bebi205-sandbox/beetles/spot-detection-beetles.h5
Epoch 2/16
85/85 [==============================] - 22s 259ms/step - loss: 0.0092 - head_loss: 0.0033 - abdomen_loss: 0.0032 - thorax_loss: 0.0027 - val_loss: 0.0576 - val_head_loss: 0.0198 - val_abdomen_loss: 0.0183 - val_thorax_loss: 0.0195
Epoch 00002: val_loss improved from 0.06329 to 0.05757, saving model to /notebooks/bebi205-sandbox/beetles/spot-detection-beetles.h5
Epoch 3/16
85/85 [==============================] - 22s 260ms/step - loss: 0.0056 - head_loss: 0.0020 - abdomen_loss: 0.0019 - thorax_loss: 0.0017 - val_loss: 0.0507 - val_head_loss: 0.0161 - val_abdomen_loss: 0.0183 - val_thorax_loss: 0.0163
Epoch 00003: val_loss improved from 0.05757 to 0.05072, saving model to /notebooks/bebi205-sandbox/beetles/spot-detection-beetles.h5
Epoch 4/16
85/85 [==============================] - 23s 265ms/step - loss: 0.0051 - head_loss: 0.0018 - abdomen_loss: 0.0019 - thorax_loss: 0.0014 - val_loss: 0.0539 - val_head_loss: 0.0182 - val_abdomen_loss: 0.0180 - val_thorax_loss: 0.0178
Epoch 00004: val_loss did not improve from 0.05072
Epoch 5/16
85/85 [==============================] - 22s 262ms/step - loss: 0.0045 - head_loss: 0.0017 - abdomen_loss: 0.0015 - thorax_loss: 0.0013 - val_loss: 0.0455 - val_head_loss: 0.0144 - val_abdomen_loss: 0.0166 - val_thorax_loss: 0.0146
Epoch 00005: val_loss improved from 0.05072 to 0.04548, saving model to /notebooks/bebi205-sandbox/beetles/spot-detection-beetles.h5
Epoch 6/16
85/85 [==============================] - 22s 264ms/step - loss: 0.0037 - head_loss: 0.0013 - abdomen_loss: 0.0013 - thorax_loss: 0.0011 - val_loss: 0.0353 - val_head_loss: 0.0117 - val_abdomen_loss: 0.0114 - val_thorax_loss: 0.0122
Epoch 00006: val_loss improved from 0.04548 to 0.03531, saving model to /notebooks/bebi205-sandbox/beetles/spot-detection-beetles.h5
Epoch 7/16
85/85 [==============================] - 22s 263ms/step - loss: 0.0034 - head_loss: 0.0013 - abdomen_loss: 0.0011 - thorax_loss: 9.7032e-04 - val_loss: 0.0381 - val_head_loss: 0.0113 - val_abdomen_loss: 0.0137 - val_thorax_loss: 0.0131
Epoch 00007: val_loss did not improve from 0.03531
Epoch 8/16
85/85 [==============================] - 23s 266ms/step - loss: 0.0032 - head_loss: 0.0011 - abdomen_loss: 0.0011 - thorax_loss: 9.0177e-04 - val_loss: 0.0258 - val_head_loss: 0.0077 - val_abdomen_loss: 0.0095 - val_thorax_loss: 0.0085
Epoch 00008: val_loss improved from 0.03531 to 0.02577, saving model to /notebooks/bebi205-sandbox/beetles/spot-detection-beetles.h5
Epoch 9/16
85/85 [==============================] - 22s 263ms/step - loss: 0.0026 - head_loss: 9.4825e-04 - abdomen_loss: 8.6283e-04 - thorax_loss: 7.4661e-04 - val_loss: 0.0117 - val_head_loss: 0.0036 - val_abdomen_loss: 0.0048 - val_thorax_loss: 0.0034
Epoch 00009: val_loss improved from 0.02577 to 0.01174, saving model to /notebooks/bebi205-sandbox/beetles/spot-detection-beetles.h5
Epoch 10/16
85/85 [==============================] - 22s 264ms/step - loss: 0.0029 - head_loss: 0.0010 - abdomen_loss: 9.8917e-04 - thorax_loss: 8.6413e-04 - val_loss: 0.0055 - val_head_loss: 0.0019 - val_abdomen_loss: 0.0021 - val_thorax_loss: 0.0015
Epoch 00010: val_loss improved from 0.01174 to 0.00547, saving model to /notebooks/bebi205-sandbox/beetles/spot-detection-beetles.h5
Epoch 11/16
85/85 [==============================] - 23s 266ms/step - loss: 0.0023 - head_loss: 8.2611e-04 - abdomen_loss: 8.1147e-04 - thorax_loss: 7.0860e-04 - val_loss: 0.0030 - val_head_loss: 0.0012 - val_abdomen_loss: 0.0011 - val_thorax_loss: 7.4795e-04
Epoch 00011: val_loss improved from 0.00547 to 0.00305, saving model to /notebooks/bebi205-sandbox/beetles/spot-detection-beetles.h5
Epoch 12/16
85/85 [==============================] - 22s 263ms/step - loss: 0.0027 - head_loss: 9.6934e-04 - abdomen_loss: 9.1597e-04 - thorax_loss: 7.8951e-04 - val_loss: 0.0028 - val_head_loss: 0.0010 - val_abdomen_loss: 0.0011 - val_thorax_loss: 7.0343e-04
Epoch 00012: val_loss improved from 0.00305 to 0.00283, saving model to /notebooks/bebi205-sandbox/beetles/spot-detection-beetles.h5
Epoch 13/16
85/85 [==============================] - 22s 263ms/step - loss: 0.0025 - head_loss: 8.9262e-04 - abdomen_loss: 8.5808e-04 - thorax_loss: 7.5898e-04 - val_loss: 0.0028 - val_head_loss: 0.0010 - val_abdomen_loss: 7.3291e-04 - val_thorax_loss: 0.0010
Epoch 00013: val_loss improved from 0.00283 to 0.00281, saving model to /notebooks/bebi205-sandbox/beetles/spot-detection-beetles.h5
Epoch 14/16
85/85 [==============================] - 22s 262ms/step - loss: 0.0023 - head_loss: 8.6177e-04 - abdomen_loss: 7.9405e-04 - thorax_loss: 6.8307e-04 - val_loss: 0.0016 - val_head_loss: 6.5850e-04 - val_abdomen_loss: 5.4169e-04 - val_thorax_loss: 4.1833e-04
Epoch 00014: val_loss improved from 0.00281 to 0.00162, saving model to /notebooks/bebi205-sandbox/beetles/spot-detection-beetles.h5
Epoch 15/16
85/85 [==============================] - 22s 262ms/step - loss: 0.0020 - head_loss: 7.1179e-04 - abdomen_loss: 6.9006e-04 - thorax_loss: 5.7563e-04 - val_loss: 0.0021 - val_head_loss: 8.0776e-04 - val_abdomen_loss: 6.9747e-04 - val_thorax_loss: 5.6267e-04
Epoch 00015: val_loss did not improve from 0.00162
Epoch 16/16
85/85 [==============================] - 23s 264ms/step - loss: 0.0020 - head_loss: 6.8609e-04 - abdomen_loss: 7.2187e-04 - thorax_loss: 5.8270e-04 - val_loss: 0.0018 - val_head_loss: 6.4838e-04 - val_abdomen_loss: 6.8829e-04 - val_thorax_loss: 5.0822e-04
Epoch 00016: val_loss did not improve from 0.00162
Generate predictions#
test_iter = iter(bug_data.test_dataset)
# Visualize predictions
import scipy.ndimage as nd
from skimage.morphology import watershed, remove_small_objects, h_maxima, disk, square, dilation, local_maxima
from skimage.feature import peak_local_max
def post_processing(transform_img):
max_list = []
transform_img = transform_img[...,0]
transform_img = nd.gaussian_filter(transform_img, 1)
maxima = peak_local_max(image=transform_img,
min_distance=5,
threshold_abs=0.25,
exclude_border=False,
indices=True)
return maxima
X_dict, y_true_dict = next(test_iter)
y_pred_list = bug_model.predict(X_dict)
head_markers = post_processing(y_pred_list[0][0,...])
abdomen_markers = post_processing(y_pred_list[1][0,...])
thorax_markers = post_processing(y_pred_list[2][0,...])
head_markers_true = post_processing(y_true_dict['head'][0,...])
abdomen_markers_true = post_processing(y_true_dict['abdomen'][0,...])
thorax_markers_true = post_processing(y_true_dict['thorax'][0,...])
print(head_markers)
print(abdomen_markers)
print(thorax_markers)
y_pred_dict = {'head':y_pred_list[0],
'abdomen':y_pred_list[1],
'thorax':y_pred_list[2]}
fig, axes = plt.subplots(2,4, figsize=(20,10))
axes[0,0].imshow(X_dict['X'][0,...], cmap='gray')
axes[0,0].scatter(head_markers[:,1], head_markers[:,0], color='c')
axes[0,0].scatter(abdomen_markers[:,1], abdomen_markers[:,0], color='m')
axes[0,0].scatter(thorax_markers[:,1], thorax_markers[:,0], color='y')
axes[0,1].imshow(y_pred_dict['head'][0,...], cmap='jet')
axes[0,2].imshow(y_pred_dict['abdomen'][0,...], cmap='jet')
axes[0,3].imshow(y_pred_dict['thorax'][0,...], cmap='jet')
axes[1,0].imshow(X_dict['X'][0,...], cmap='gray')
axes[1,0].scatter(head_markers_true[:,1], head_markers_true[:,0], color='c')
axes[1,0].scatter(abdomen_markers_true[:,1], abdomen_markers_true[:,0], color='m')
axes[1,0].scatter(thorax_markers_true[:,1], thorax_markers_true[:,0], color='y')
axes[1,1].imshow(y_true_dict['head'][0,...], cmap='jet')
axes[1,2].imshow(y_true_dict['abdomen'][0,...], cmap='jet')
axes[1,3].imshow(y_true_dict['thorax'][0,...], cmap='jet')
[[163 156]
[ 85 127]]
[[ 65 103]
[143 163]]
[[159 158]
[ 81 120]]
<matplotlib.image.AxesImage at 0x7fab20e87ef0>
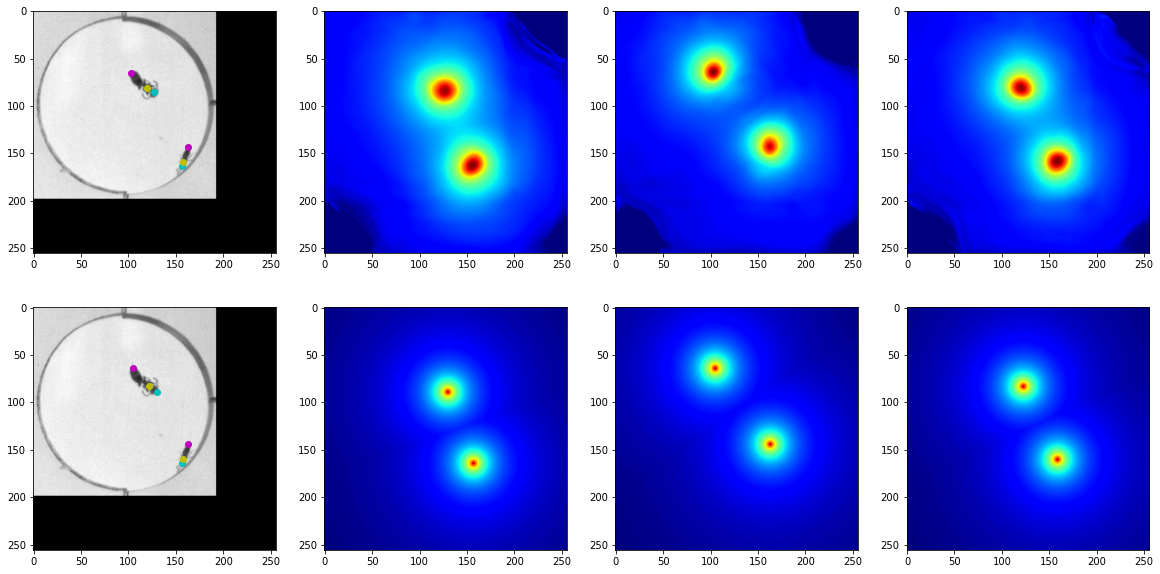
Benchmark model performance#
Collect a set of samples from the test dataset in order to benchmark model performance.
y_true, y_pred = [], []
for X_dict, y_true_dict in bug_data.test_dataset:
y_pred_list = bug_model.predict(X_dict)
# Extract predicted points
y_pred.append(post_processing(y_pred_list[0][0,...]))
y_pred.append(post_processing(y_pred_list[1][0,...]))
y_pred.append(post_processing(y_pred_list[2][0,...]))
# Extract true points from transformed images
y_true.append(post_processing(y_true_dict['head'][0,...]))
y_true.append(post_processing(y_true_dict['abdomen'][0,...]))
y_true.append(post_processing(y_true_dict['thorax'][0,...]))
y_true = np.concatenate(y_true)
y_pred = np.concatenate(y_pred)
from scipy.optimize import linear_sum_assignment
from scipy.spatial.distance import cdist
import scipy.spatial
def sum_of_min_distance(pts1, pts2, normalized=False):
"""Calculates the sum of minimal distance measure between two sets of d-dimensional points
as suggested by Eiter and Mannila in:
https://link.springer.com/article/10.1007/s002360050075
Args:
pts1 ((N1,d) numpy.array): set of N1 points in d dimensions
pts2 ((N2,d) numpy.array): set of N2 points in d dimensions
each row of pts1 and pts2 should be the coordinates of a single d-dimensional point
normalized (bool): if true, each sum will be normalized by the number of elements in it,
resulting in an intensive distance measure which doesn't scale like the number of points
Returns:
float: the sum of minimal distance between point sets X and Y, defined as:
d(X,Y) = 1/2 * (sum over x in X of min on y in Y of d(x,y)
+ sum over y in Y of min on x in X of d(x,y))
= 1/2( sum over x in X of d(x,Y) + sum over y in Y of d(X,y))
where d(x,y) is the Euclidean distance
Note that this isn't a metric in the mathematical sense (it doesn't satisfy the triangle
inequality)
"""
if len(pts1) == 0 or len(pts2) == 0:
return np.inf
# for each point in each of the sets, find its nearest neighbor from the other set
tree1 = scipy.spatial.cKDTree(pts1, leafsize=2)
dist21, _ = tree1.query(pts2)
tree2 = scipy.spatial.cKDTree(pts2, leafsize=2)
dist12, _ = tree2.query(pts1)
if normalized:
d_md = 0.5 * (np.mean(dist21) + np.mean(dist12))
else:
d_md = 0.5 * (np.sum(dist21) + np.sum(dist12))
return d_md
def match_points_mutual_nearest_neighbor(pts1, pts2, threshold=None):
'''Find a pairing between two sets of points that ensures that each pair of points are mutual nearest neighbors.
Args:
pts1 ((N1,d) numpy.array): a set of N1 points in d dimensions
pts2 ((N2,d) numpy.array): a set of N2 points in d dimensions
where N1/N2 is the number of points and d is the dimension
threshold (float): a distance threshold for matching two points. Points that are more than the threshold
distance apart, cannot be matched
Returns:
row_ind, col_ind (arrays):
An array of row indices and one of corresponding column indices giving the optimal assignment, as described in:
https://docs.scipy.org/doc/scipy/reference/generated/scipy.optimize.linear_sum_assignment.html
'''
# calculate the distances between true points and their nearest predicted points
# and the distances between predicted points and their nearest true points
tree1 = scipy.spatial.cKDTree(pts1, leafsize=2)
dist_to_nearest1, nearest_ind1 = tree1.query(pts2)
# dist_to_nearest1[i] is the distance between pts1 point i and the pts2 point closest to it
# nearest_ind1[i] is equal to j if pts1[j] is the nearest to pts2[i] from all of pts1
tree2 = scipy.spatial.cKDTree(pts2, leafsize=2)
dist_to_nearest2, nearest_ind2 = tree2.query(pts1)
# dist_to_nearest2[i] is the distance between pts2 point i and the pts1 point nearest to it
# nearest_ind2[i] is equal to j if pts2[j] is the nearest to pts1[i] from all of pts2
# calculate the number of true positives
pt_has_mutual_nn2 = nearest_ind2[nearest_ind1] == list(range(len(nearest_ind1)))
pt_has_mutual_nn1 = nearest_ind1[nearest_ind2] == list(range(len(nearest_ind2)))
if threshold is None:
row_ind = np.where(pt_has_mutual_nn1)[0]
col_ind = nearest_ind2[pt_has_mutual_nn1]
else:
pt_close_enough_to_nn1 = dist_to_nearest2 <= threshold
matched_pts1 = pt_has_mutual_nn1 & pt_close_enough_to_nn1
col_ind = nearest_ind2[matched_pts1]
row_ind = np.where(matched_pts1)[0]
return row_ind, col_ind
def point_precision(points_true, points_pred, threshold, match_points_function=match_points_mutual_nearest_neighbor):
""" Calculates the precision, tp/(tp + fp), of point detection using the following definitions:
true positive (tp) = a predicted dot p with a matching true dot t,
where the matching between predicted and true points is such that the total distance between matched points is
minimized, and points can be matched only if the distance between them is smaller than the threshold.
Otherwise, the predicted dot is a false positive (fp).
The precision is equal to (the number of true positives) / (total number of predicted points)
Args:
points_true ((N1,d) numpy.array): ground truth points for a single image
points_pred ((N2,d) numpy.array): predicted points for a single image
where N1/N2 is the number of points and d is the dimension
threshold (float): a distance threshold used in the definition of tp and fp
match_points_function: a function that matches points in two sets,
and has three parameters: pts1, pts2, threshold -
two sets of points, and a threshold distance for allowing a match
supported matching functions are match_points_min_dist, match_points_mutual_nearest_neighbor
Returns:
float: the precision as defined above (a number between 0 and 1)
"""
if len(points_true) == 0 or len(points_pred) == 0:
return 0
# find the minimal sum of distances matching between the points
row_ind, col_ind = match_points_function(points_true, points_pred, threshold=threshold)
# number of true positives = number of pairs matched
tp = len(row_ind)
precision = tp / len(points_pred)
return precision
def point_recall(points_true, points_pred, threshold, match_points_function=match_points_mutual_nearest_neighbor):
"""Calculates the recall, tp/(tp + fn), of point detection using the following definitions:
true positive (tp) = a predicted dot p with a matching true dot t,
where the matching between predicted and true points is such that the total distance between matched points is
minimized, and points can be matched only if the distance between them is smaller than the threshold.
Otherwise, the predicted dot is a false positive (fp).
The recall is equal to (the number of true positives) / (total number of true points)
Args:
points_true ((N1,d) numpy.array): ground truth points for a single image
points_pred ((N2,d) numpy.array): predicted points for a single image
where N1/N2 is the number of points and d is the dimension
threshold (float): a distance threshold used in the definition of tp and fp
Returns:
float: the recall as defined above (a number between 0 and 1)
"""
if len(points_true) == 0 or len(points_pred) == 0:
return 0
# find the minimal sum of distances matching between the points
row_ind, col_ind = match_points_function(points_true, points_pred, threshold=threshold)
# number of true positives = number of pairs matched
tp = len(row_ind)
recall = tp / len(points_true)
return recall
def point_F1_score(points_true, points_pred, threshold, match_points_function=match_points_mutual_nearest_neighbor):
"""Calculates the F1 score of dot detection using the following definitions:
F1 score = 2*p*r / (p+r)
where
p = precision = (the number of true positives) / (total number of predicted points)
r = recall = (the number of true positives) / (total number of true points)
and
true positive (tp) = a predicted dot p with a matching true dot t,
where the matching between predicted and true points is such that the total distance between matched points is
minimized, and points can be matched only if the distance between them is smaller than the threshold.
Otherwise, the predicted dot is a false positive (fp).
Args:
points_true ((N1,d) numpy.array): ground truth points for a single image
points_pred ((N2,d) numpy.array): predicted points for a single image
where N1/N2 is the number of points and d is the dimension
threshold (float): a distance threshold used in the definition of tp and fp
Returns:
float: the F1 score as defined above (a number between 0 and 1)
"""
p = point_precision(points_true, points_pred, threshold)
r = point_recall(points_true, points_pred, threshold)
if p == 0 or r == 0:
return 0
F1 = 2 * p * r / (p + r)
return F1
def stats_points(points_true, points_pred, threshold, match_points_function=match_points_mutual_nearest_neighbor):
"""Calculates point-based statistics
(precision, recall, F1, JAC, RMSE, d_md)
Args:
points_true ((N1,d) numpy.array): ground truth points for a single image
points_pred ((N2,d) numpy.array): predicted points for a single image
where N1/N2 is the number of points and d is the dimension
threshold (float): a distance threshold used in the definition of tp and fp
Returns:
dictionary: containing the calculated statistics
"""
# if one of the point sets is empty, precision=recall=0
if len(points_true) == 0 or len(points_pred) == 0:
p = 0
r = 0
F1 = 0
J = 0
RMSE = None
dmd = None
return {
'precision': p,
'recall': r,
'F1': F1,
'JAC': J,
'RMSE': RMSE,
'd_md': dmd
}
# find the minimal sum of distances matching between the points
row_ind, col_ind = match_points_function(points_true, points_pred, threshold=threshold)
# number of true positives = number of pairs matched
tp = len(row_ind)
p = tp / len(points_pred)
r = tp / len(points_true)
# calculate the F1 score from the precision and the recall
if p == 0 or r == 0:
F1 = 0
else:
F1 = 2*p*r / (p+r)
# calculate the Jaccard index from the F1 score
J = F1 / (2 - F1)
# calculate the RMSE for matched pairs
if len(row_ind) == 0:
RMSE = None
d_md = None
else:
dist_sq_sum = np.sum(np.sum((points_true[row_ind] - points_pred[col_ind]) ** 2, axis=1))
RMSE = np.sqrt(dist_sq_sum/len(row_ind)/2)
# RMSE = np.sqrt(mean_squared_error(points_true[row_ind], points_pred[col_ind]))
# calculate the mean sum to nearest neighbor from other set
d_md = sum_of_min_distance(points_true[row_ind], points_pred[col_ind], normalized=True)
return {
'precision': p,
'recall': r,
'F1': F1,
'Jaccard Index': J,
'Root Mean Squared Error': RMSE,
'Average Distance': d_md
}
stats_points(y_true, y_pred, 20)
{'precision': 0.9361702127659575,
'recall': 0.7457627118644068,
'F1': 0.8301886792452831,
'Jaccard Index': 0.7096774193548387,
'Root Mean Squared Error': 1.8799177931736561,
'Average Distance': 2.241467514540121}
%load_ext watermark
%watermark -u -d -vm --iversions
re 2.2.1
pandas 1.1.5
skimage 0.17.2
numpy 1.19.5
tensorflow_addons 0.12.1
scipy.ndimage 2.0
tensorflow 2.4.1
sklearn 0.24.1
imageio 2.9.0
last updated: 2021-04-27
CPython 3.6.9
IPython 7.16.1
compiler : GCC 8.4.0
system : Linux
release : 4.15.0-142-generic
machine : x86_64
processor : x86_64
CPU cores : 24
interpreter: 64bit