Image classification with TensorFlow#
In this notebook we will revisit the image classification problem with TensorFLow. You will see how to create models and how to access the machinery of stochastic gradient descent using the existing software tooling.
Load packages#
In this cell, we will load the python packages we need for this notebook
!pip install tensorflow-addons
import imageio
import skimage
import sklearn.model_selection
import skimage.color
import skimage.transform
import pandas as pd
import numpy as np
import matplotlib.pyplot as plt
import tensorflow as tf
gpus = tf.config.experimental.list_physical_devices('GPU')
for gpu in gpus:
tf.config.experimental.set_memory_growth(gpu, True)
import tensorflow_addons as tfa
import os
The supervised machine learning workflow#
Recall the workflow outlined in the linear classifier notebook
Create a training dataset
Specify a model
Specify a loss function, optimization algorithm, and training parameters
Train the model
Benchmark the model We will implement these steps using TensorFlow in this notebook
Create training data#
We will reuse our toy example of building a classifier that can distinguish cats from dogs. The dataset that we are working with is a subset of Kaggle’s Dogs vs. Cats dataset.
!wget https://storage.googleapis.com/datasets-spring2021/cats-and-dogs.npz
# Load data from the downloaded npz file
with np.load('cats-and-dogs.npz') as f:
X = f['X']
y = f['y']
print(X.shape, y.shape)
(8192, 128, 128, 3) (8192,)
# Visualize a collection of images in the training data
fig, axes = plt.subplots(4, 2, figsize=(20,20))
for i in range(8):
axes.flatten()[i].imshow(X[i,...,:])
axes.flatten()[i].set_title('Label ' + str(y[i]))
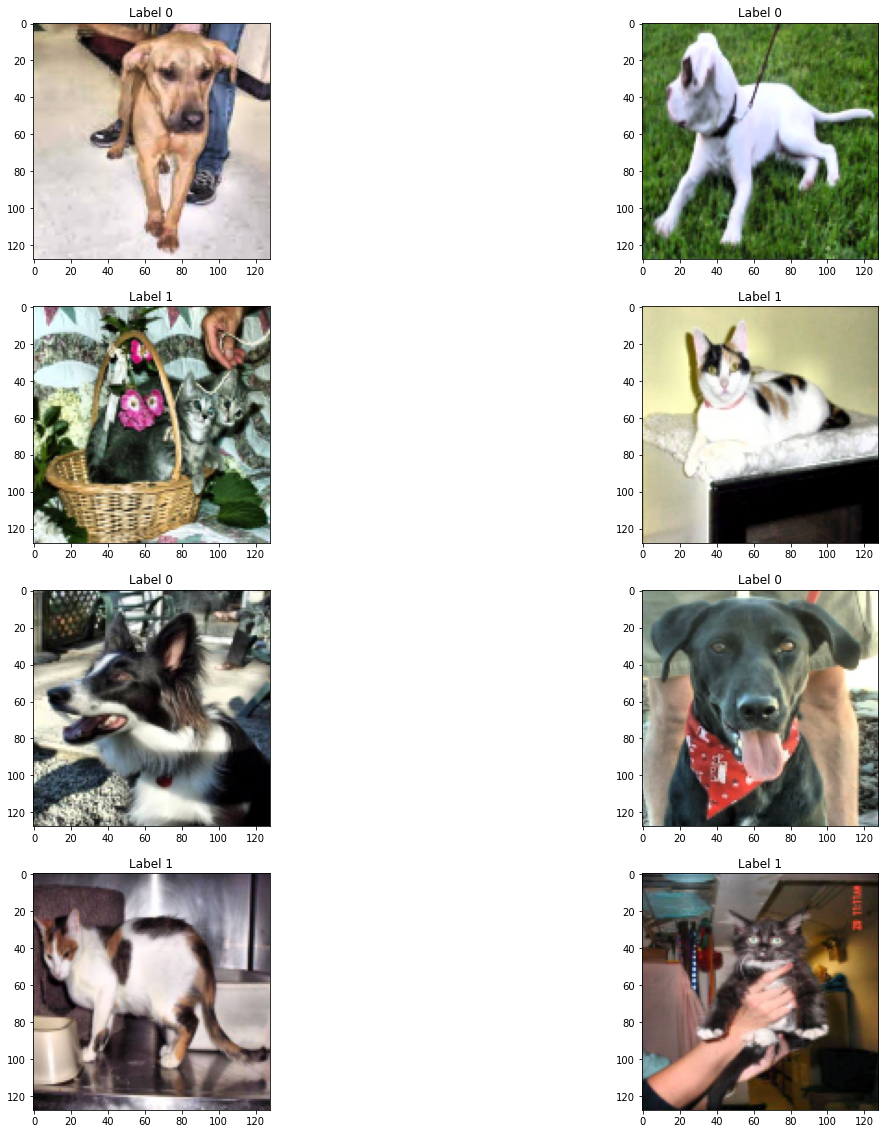
Create dataset object#
TensorFlow uses Dataset objects to feed data into the training pipeline. These objects were covered in more detail in the TensorFlow Dataset notebook. In this section, we will make a class that builds a dataset object and applies random augmentation operation (e.g. rotation, flipping, scaling).
# Create dataset builder
class DatasetBuilder(object):
def __init__(self,
X,
y,
batch_size=1,
rotation_range=180,
scale_range=(0.75, 1.25)):
self.X = X
self.y = tf.keras.utils.to_categorical(y)
self.batch_size = batch_size
self.rotation_range = np.float(rotation_range)
self.scale_range = scale_range
# Create dataset
self._create_dataset()
def _augment(self, *args):
img = args[0]
label = args[1]
theta = tf.random.uniform([1], 0, 2*np.pi*self.rotation_range/360)
img = tfa.image.rotate(img, theta)
img = tf.image.random_flip_left_right(img)
img = tf.image.random_flip_up_down(img)
return (img, label)
def _create_dataset(self):
X_train, X_temp, y_train, y_temp = sklearn.model_selection.train_test_split(self.X, self.y, train_size=0.8)
X_val, X_test, y_val, y_test = sklearn.model_selection.train_test_split(X_temp, y_temp, train_size=0.5)
train_dataset = tf.data.Dataset.from_tensor_slices((X_train, y_train))
val_dataset = tf.data.Dataset.from_tensor_slices((X_val, y_val))
test_dataset = tf.data.Dataset.from_tensor_slices((X_test, y_test))
self.train_dataset = train_dataset.shuffle(256).batch(self.batch_size).map(self._augment)
self.val_dataset = val_dataset.batch(self.batch_size)
self.test_dataset = test_dataset.batch(self.batch_size)
db = DatasetBuilder(X, y, batch_size=64)
# Check the data augmentation
it = db.train_dataset.as_numpy_iterator()
X_temp, y_temp = it.next()
print(X_temp.shape)
fig, axes = plt.subplots(4, figsize=(20,20))
for i in range(4):
axes.flatten()[i].imshow(X_temp[i,...,:])
axes.flatten()[i].set_title('Label ' + str(y_temp[i]))
(64, 128, 128, 3)
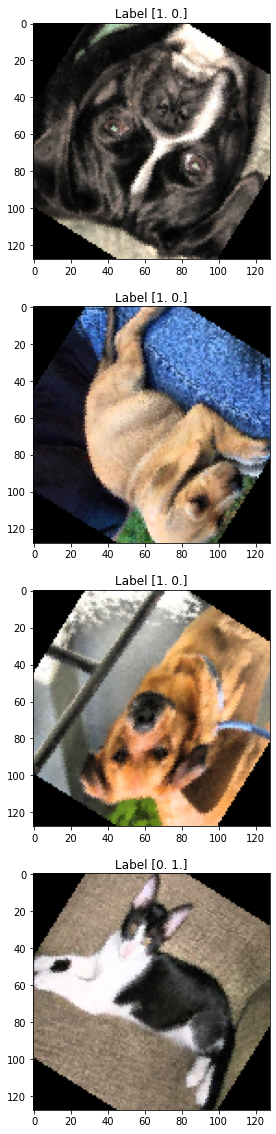
Define models#
In this section, we will define our machine learning models using TensorFlow. These models are composed of layers - each layer specifies a mathematical operation that is applied to its input. The nice thing about TensorFlow is that almost all of the machinery required for stochastic gradient descent is taken care of for us.
Specify trainable variables? Check.
Initialize trainable variables with random values? Check.
Compute the layer outputs? Check.
Compute gradients using backpropagation? Check.
Perform all of the computations on GPUs to speed up training and inference? Check. All of the above (and more) are taken care of for us by TensorFlow - writing models often requires little math (although one practice that I encourage is keeping track of the input and output dimensions for each layer).
Let define three different models so that we can evaluate their performance on this problem.
Linear classifier
Fully connected neural network
Convolutional neural network (a simple one)
To define these models, we will use a module in TensorFlow called Keras. Keras simple APIs for specifying models. In Keras, there are two different APIs you can use:
Sequential API - If your model is composed of a linear sequence of steps, this is the easier API to use.
Functional API - If your model is more complicated, this API provides more flexibility. If you’re using the functional API, consider using a class with methods to write submodels. The TensorFlow documentation provides additional details about how to use each of these two APIs.
from tensorflow.keras.layers import Input, Flatten, Dense, Activation, BatchNormalization, Conv2D, MaxPool2D, Softmax
from tensorflow.keras import Model
from tensorflow.keras.utils import plot_model
# Define the linear classifier
def create_linear_classifier():
inputs = Input((IMG_HEIGHT, IMG_WIDTH, 3),
name='linear_classifier_input')
x = Flatten()(inputs)
x = Dense(2)(x)
x = Softmax(axis=-1)(x)
model = Model(inputs=inputs, outputs=x)
return model
linear_classifier = create_linear_classifier()
plot_model(linear_classifier, show_shapes=True)
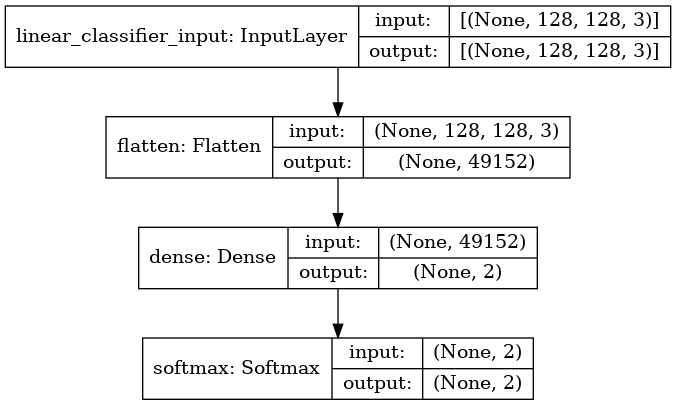
# Define the fully connected neural network
def create_fc_classifier():
inputs = Input((IMG_HEIGHT, IMG_WIDTH, 3),
name='fc_classifier_input')
x = Flatten()(inputs)
x = Dense(64)(x)
x = Activation('relu')(x)
x = BatchNormalization(axis=-1)(x)
x = Dense(64)(x)
x = Activation('relu')(x)
x = BatchNormalization(axis=-1)(x)
x = Dense(2)(x)
x = Softmax(axis=-1)(x)
model = Model(inputs=inputs, outputs=x)
return model
fc_classifier = create_fc_classifier()
plot_model(fc_classifier, show_shapes=True)
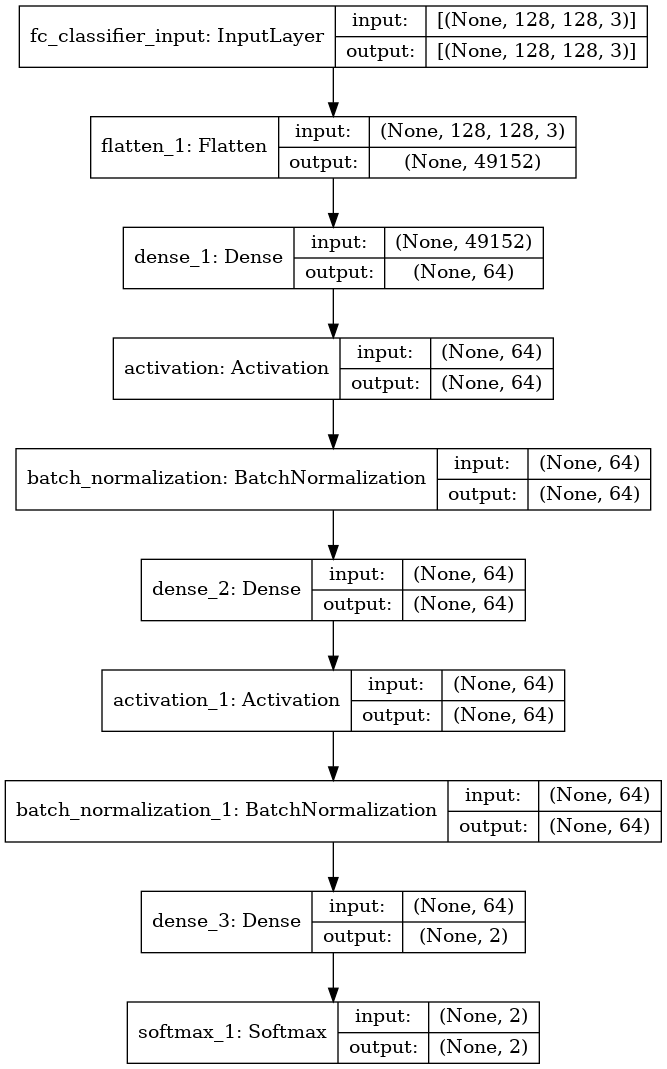
# Define the convolutional neural network
def create_conv_classifier():
inputs = Input((IMG_HEIGHT, IMG_WIDTH, 3),
name='conv_classifier_input')
x = Conv2D(64, (3,3), padding='SAME')(inputs)
x = Activation('relu')(x)
x = BatchNormalization(axis=-1)(x)
x = MaxPool2D(strides=(2,2))(x) # 16, 16
x = Conv2D(64, (3,3), padding='SAME')(x)
x = Activation('relu')(x)
x = BatchNormalization(axis=-1)(x)
x = MaxPool2D(strides=(2,2))(x) # 8,8
x = Conv2D(64, (3,3), padding='SAME')(x)
x = Activation('relu')(x)
x = BatchNormalization(axis=-1)(x)
x = MaxPool2D(strides=(2,2))(x) # 4,4
x = Conv2D(64, (3,3), padding='SAME')(x)
x = Activation('relu')(x)
x = BatchNormalization(axis=-1)(x)
x = MaxPool2D(strides=(2,2))(x) # 2,2
x = Flatten()(x)
x = Dense(2)(x)
x = Softmax(axis=-1)(x)
model = Model(inputs=inputs, outputs=x)
return model
conv_classifier = create_conv_classifier()
plot_model(conv_classifier, show_shapes=True)
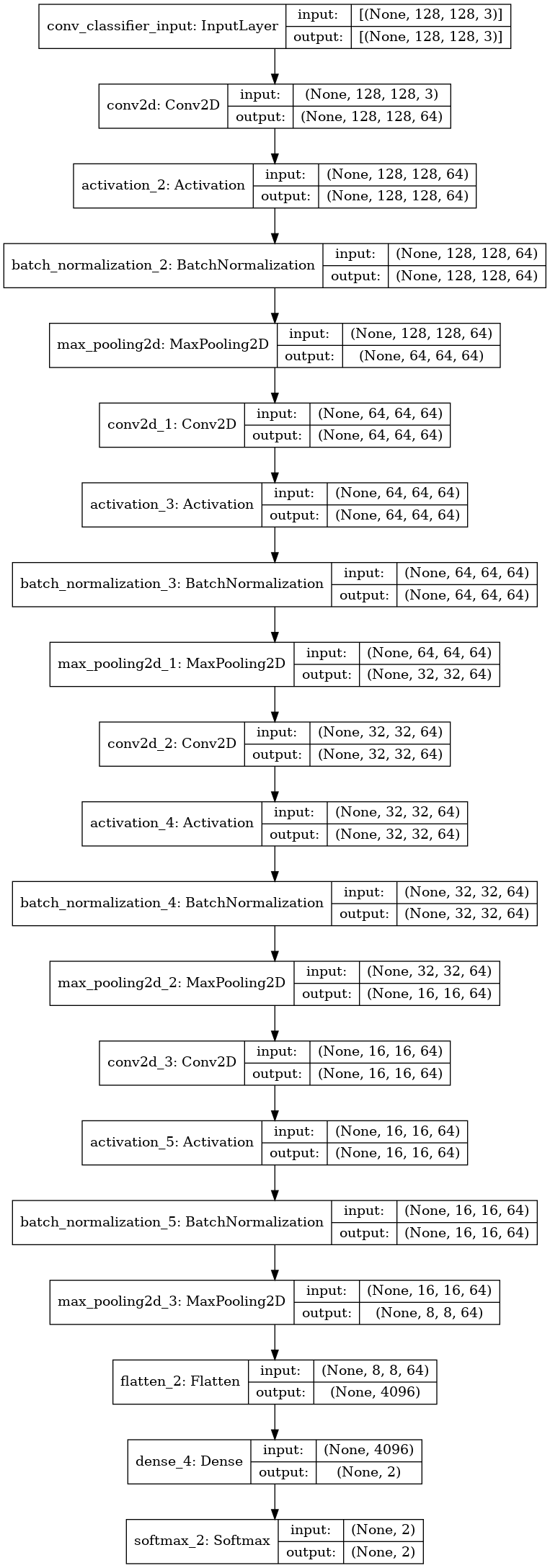
Specify training parameters#
In this section, we will specify how we want to train the neural network. We will need to specify three things:
The loss function. Because we are training a model for classification, we will use the categorical crossentropy
The training algorithm. There are many flavors of stochastic gradient descent - for this problem, we will use a variant called Adam
The training parameters. The training algorithm needs parameters like the learning rate, number of epochs, number of steps per epoch, etc. to be specified
# Define the loss function
loss_function = tf.keras.losses.CategoricalCrossentropy()
# Define the training algorithm
linear_optimizer = tf.keras.optimizers.Adam(lr=1e-3, clipnorm=0.001)
fc_optimizer = tf.keras.optimizers.Adam(lr=1e-3, clipnorm=0.001)
conv_optimizer = tf.keras.optimizers.Adam(lr=1e-3, clipnorm=0.001)
# Define training parameters
training_steps_per_epoch=512
n_epochs=32
# Define callbacks
linear_model_path = '/data/models/bebi205/linear'
linear_callbacks = [
tf.keras.callbacks.ModelCheckpoint(
linear_model_path, monitor='val_loss',
save_best_only=True, verbose=1,
save_weights_only=False)
]
linear_callbacks.append(
tf.keras.callbacks.ReduceLROnPlateau(
monitor='val_loss', factor=0.5, verbose=1,
patience=3, min_lr=1e-7)
)
fc_model_path = '/data/models/bebi205/fc'
fc_callbacks = [
tf.keras.callbacks.ModelCheckpoint(
fc_model_path, monitor='val_loss',
save_best_only=True, verbose=1,
save_weights_only=False)
]
fc_callbacks.append(
tf.keras.callbacks.ReduceLROnPlateau(
monitor='val_loss', factor=0.5, verbose=1,
patience=3, min_lr=1e-7)
)
conv_model_path = '/data/models/bebi205/conv'
conv_callbacks = [
tf.keras.callbacks.ModelCheckpoint(
conv_model_path, monitor='val_loss',
save_best_only=True, verbose=1,
save_weights_only=False)
]
conv_callbacks.append(
tf.keras.callbacks.ReduceLROnPlateau(
monitor='val_loss', factor=0.5, verbose=1,
patience=3, min_lr=1e-7)
)
# Define metrics
recall_0 = tf.keras.metrics.Recall(class_id=0)
recall_1 = tf.keras.metrics.Recall(class_id=1)
precision_0 = tf.keras.metrics.Precision(class_id=0)
precision_1 = tf.keras.metrics.Precision(class_id=1)
# Compile models
linear_classifier.compile(optimizer=linear_optimizer,
loss=loss_function,
metrics = [recall_0, recall_1, precision_0, precision_1])
fc_classifier.compile(optimizer=fc_optimizer,
loss=loss_function,
metrics = [recall_0, recall_1, precision_0, precision_1])
conv_classifier.compile(optimizer=conv_optimizer,
loss=loss_function,
metrics = [recall_0, recall_1, precision_0, precision_1])
Train the model#
With the dataset, model, and training parameters defined, it is straightforward to train a model. Keras Model objects have a fit method that takes in the training parameters and executes the training algorithm.
# Train the linear classifier
linear_classifier.fit(db.train_dataset,
validation_data=db.val_dataset,
epochs=n_epochs,
verbose=1,
callbacks=linear_callbacks)
Epoch 1/32
103/103 [==============================] - 17s 152ms/step - loss: 3.7118 - recall: 0.5115 - recall_1: 0.5110 - precision: 0.5148 - precision_1: 0.5070 - val_loss: 1.2333 - val_recall: 0.9257 - val_recall_1: 0.1269 - val_precision: 0.5237 - val_precision_1: 0.6220
Epoch 00001: val_loss improved from inf to 1.23329, saving model to /data/models/bebi205/linear
INFO:tensorflow:Assets written to: /data/models/bebi205/linear/assets
Epoch 2/32
103/103 [==============================] - 15s 146ms/step - loss: 2.9236 - recall: 0.4683 - recall_1: 0.5180 - precision: 0.4985 - precision_1: 0.4876 - val_loss: 1.0794 - val_recall: 0.8321 - val_recall_1: 0.2463 - val_precision: 0.5338 - val_precision_1: 0.5858
Epoch 00002: val_loss improved from 1.23329 to 1.07944, saving model to /data/models/bebi205/linear
INFO:tensorflow:Assets written to: /data/models/bebi205/linear/assets
Epoch 3/32
103/103 [==============================] - 15s 144ms/step - loss: 3.3565 - recall: 0.5535 - recall_1: 0.4897 - precision: 0.5292 - precision_1: 0.5148 - val_loss: 1.7708 - val_recall: 0.9161 - val_recall_1: 0.1070 - val_precision: 0.5155 - val_precision_1: 0.5513
Epoch 00003: val_loss did not improve from 1.07944
Epoch 4/32
103/103 [==============================] - 15s 146ms/step - loss: 3.4448 - recall: 0.5545 - recall_1: 0.4540 - precision: 0.5117 - precision_1: 0.4957 - val_loss: 2.5203 - val_recall: 0.0408 - val_recall_1: 0.9303 - val_precision: 0.3778 - val_precision_1: 0.4832
Epoch 00004: val_loss did not improve from 1.07944
Epoch 5/32
103/103 [==============================] - 15s 146ms/step - loss: 3.3331 - recall: 0.5061 - recall_1: 0.5592 - precision: 0.5215 - precision_1: 0.5285 - val_loss: 5.1110 - val_recall: 0.0048 - val_recall_1: 0.9975 - val_precision: 0.6667 - val_precision_1: 0.4914
Epoch 00005: val_loss did not improve from 1.07944
Epoch 00005: ReduceLROnPlateau reducing learning rate to 0.0005000000237487257.
Epoch 6/32
103/103 [==============================] - 15s 146ms/step - loss: 3.6237 - recall: 0.4926 - recall_1: 0.5285 - precision: 0.5059 - precision_1: 0.5041 - val_loss: 0.8672 - val_recall: 0.7002 - val_recall_1: 0.3831 - val_precision: 0.5407 - val_precision_1: 0.5520
Epoch 00006: val_loss improved from 1.07944 to 0.86725, saving model to /data/models/bebi205/linear
INFO:tensorflow:Assets written to: /data/models/bebi205/linear/assets
Epoch 7/32
103/103 [==============================] - 15s 144ms/step - loss: 1.7365 - recall: 0.5338 - recall_1: 0.4838 - precision: 0.5135 - precision_1: 0.5031 - val_loss: 2.8970 - val_recall: 0.9784 - val_recall_1: 0.0721 - val_precision: 0.5224 - val_precision_1: 0.7632
Epoch 00007: val_loss did not improve from 0.86725
Epoch 8/32
103/103 [==============================] - 15s 144ms/step - loss: 2.3155 - recall: 0.5537 - recall_1: 0.4833 - precision: 0.5180 - precision_1: 0.5161 - val_loss: 1.1853 - val_recall: 0.1775 - val_recall_1: 0.8930 - val_precision: 0.6325 - val_precision_1: 0.5114
Epoch 00008: val_loss did not improve from 0.86725
Epoch 9/32
103/103 [==============================] - 15s 144ms/step - loss: 2.2149 - recall: 0.4894 - recall_1: 0.5226 - precision: 0.5043 - precision_1: 0.5024 - val_loss: 2.6514 - val_recall: 0.0336 - val_recall_1: 0.9876 - val_precision: 0.7368 - val_precision_1: 0.4963
Epoch 00009: val_loss did not improve from 0.86725
Epoch 00009: ReduceLROnPlateau reducing learning rate to 0.0002500000118743628.
Epoch 10/32
103/103 [==============================] - 15s 145ms/step - loss: 1.3123 - recall: 0.5462 - recall_1: 0.5158 - precision: 0.5357 - precision_1: 0.5266 - val_loss: 0.8366 - val_recall: 0.4341 - val_recall_1: 0.6866 - val_precision: 0.5896 - val_precision_1: 0.5391
Epoch 00010: val_loss improved from 0.86725 to 0.83658, saving model to /data/models/bebi205/linear
INFO:tensorflow:Assets written to: /data/models/bebi205/linear/assets
Epoch 11/32
103/103 [==============================] - 15s 146ms/step - loss: 1.2420 - recall: 0.5201 - recall_1: 0.5421 - precision: 0.5337 - precision_1: 0.5295 - val_loss: 1.5222 - val_recall: 0.9928 - val_recall_1: 0.0323 - val_precision: 0.5156 - val_precision_1: 0.8125
Epoch 00011: val_loss did not improve from 0.83658
Epoch 12/32
103/103 [==============================] - 15s 144ms/step - loss: 0.9674 - recall: 0.5593 - recall_1: 0.5436 - precision: 0.5608 - precision_1: 0.5414 - val_loss: 1.3885 - val_recall: 0.9664 - val_recall_1: 0.0945 - val_precision: 0.5254 - val_precision_1: 0.7308
Epoch 00012: val_loss did not improve from 0.83658
Epoch 13/32
103/103 [==============================] - 15s 143ms/step - loss: 1.0038 - recall: 0.5183 - recall_1: 0.5755 - precision: 0.5573 - precision_1: 0.5389 - val_loss: 0.8689 - val_recall: 0.2710 - val_recall_1: 0.8035 - val_precision: 0.5885 - val_precision_1: 0.5152
Epoch 00013: val_loss did not improve from 0.83658
Epoch 00013: ReduceLROnPlateau reducing learning rate to 0.0001250000059371814.
Epoch 14/32
103/103 [==============================] - 15s 145ms/step - loss: 0.8831 - recall: 0.5352 - recall_1: 0.5572 - precision: 0.5602 - precision_1: 0.5395 - val_loss: 1.3397 - val_recall: 0.9760 - val_recall_1: 0.0647 - val_precision: 0.5198 - val_precision_1: 0.7222
Epoch 00014: val_loss did not improve from 0.83658
Epoch 15/32
103/103 [==============================] - 15s 144ms/step - loss: 0.8244 - recall: 0.5923 - recall_1: 0.5287 - precision: 0.5622 - precision_1: 0.5602 - val_loss: 1.0307 - val_recall: 0.9760 - val_recall_1: 0.0896 - val_precision: 0.5265 - val_precision_1: 0.7826
Epoch 00015: val_loss did not improve from 0.83658
Epoch 16/32
103/103 [==============================] - 15s 145ms/step - loss: 0.8284 - recall: 0.5942 - recall_1: 0.5043 - precision: 0.5519 - precision_1: 0.5482 - val_loss: 0.8940 - val_recall: 0.9472 - val_recall_1: 0.1219 - val_precision: 0.5281 - val_precision_1: 0.6901
Epoch 00016: val_loss did not improve from 0.83658
Epoch 00016: ReduceLROnPlateau reducing learning rate to 6.25000029685907e-05.
Epoch 17/32
103/103 [==============================] - 15s 143ms/step - loss: 0.8261 - recall: 0.5510 - recall_1: 0.5352 - precision: 0.5487 - precision_1: 0.5378 - val_loss: 0.6908 - val_recall: 0.5683 - val_recall_1: 0.6219 - val_precision: 0.6093 - val_precision_1: 0.5814
Epoch 00017: val_loss improved from 0.83658 to 0.69084, saving model to /data/models/bebi205/linear
INFO:tensorflow:Assets written to: /data/models/bebi205/linear/assets
Epoch 18/32
103/103 [==============================] - 15s 144ms/step - loss: 0.7726 - recall: 0.5008 - recall_1: 0.6172 - precision: 0.5753 - precision_1: 0.5499 - val_loss: 0.8203 - val_recall: 0.9353 - val_recall_1: 0.1294 - val_precision: 0.5270 - val_precision_1: 0.6582
Epoch 00018: val_loss did not improve from 0.69084
Epoch 19/32
103/103 [==============================] - 15s 145ms/step - loss: 0.7071 - recall: 0.5718 - recall_1: 0.6011 - precision: 0.5936 - precision_1: 0.5800 - val_loss: 0.8310 - val_recall: 0.1703 - val_recall_1: 0.9179 - val_precision: 0.6827 - val_precision_1: 0.5161
Epoch 00019: val_loss did not improve from 0.69084
Epoch 20/32
103/103 [==============================] - 15s 145ms/step - loss: 0.7644 - recall: 0.5751 - recall_1: 0.5404 - precision: 0.5654 - precision_1: 0.5564 - val_loss: 0.7176 - val_recall: 0.8225 - val_recall_1: 0.2861 - val_precision: 0.5444 - val_precision_1: 0.6085
Epoch 00020: val_loss did not improve from 0.69084
Epoch 00020: ReduceLROnPlateau reducing learning rate to 3.125000148429535e-05.
Epoch 21/32
103/103 [==============================] - 15s 145ms/step - loss: 0.6973 - recall: 0.5646 - recall_1: 0.6071 - precision: 0.5954 - precision_1: 0.5771 - val_loss: 0.6716 - val_recall: 0.5827 - val_recall_1: 0.5597 - val_precision: 0.5786 - val_precision_1: 0.5639
Epoch 00021: val_loss improved from 0.69084 to 0.67161, saving model to /data/models/bebi205/linear
INFO:tensorflow:Assets written to: /data/models/bebi205/linear/assets
Epoch 22/32
103/103 [==============================] - 15s 145ms/step - loss: 0.7052 - recall: 0.5705 - recall_1: 0.5716 - precision: 0.5762 - precision_1: 0.5663 - val_loss: 0.7807 - val_recall: 0.9400 - val_recall_1: 0.1194 - val_precision: 0.5255 - val_precision_1: 0.6575
Epoch 00022: val_loss did not improve from 0.67161
Epoch 23/32
103/103 [==============================] - 15s 146ms/step - loss: 0.6964 - recall: 0.5959 - recall_1: 0.5651 - precision: 0.5854 - precision_1: 0.5760 - val_loss: 0.7569 - val_recall: 0.9233 - val_recall_1: 0.1393 - val_precision: 0.5267 - val_precision_1: 0.6364
Epoch 00023: val_loss did not improve from 0.67161
Epoch 24/32
103/103 [==============================] - 15s 145ms/step - loss: 0.6983 - recall: 0.6113 - recall_1: 0.5561 - precision: 0.5866 - precision_1: 0.5850 - val_loss: 0.7248 - val_recall: 0.2734 - val_recall_1: 0.8682 - val_precision: 0.6826 - val_precision_1: 0.5353
Epoch 00024: val_loss did not improve from 0.67161
Epoch 00024: ReduceLROnPlateau reducing learning rate to 1.5625000742147677e-05.
Epoch 25/32
103/103 [==============================] - 15s 145ms/step - loss: 0.7024 - recall: 0.5287 - recall_1: 0.6145 - precision: 0.5845 - precision_1: 0.5629 - val_loss: 0.7134 - val_recall: 0.8897 - val_recall_1: 0.1915 - val_precision: 0.5330 - val_precision_1: 0.6260
Epoch 00025: val_loss did not improve from 0.67161
Epoch 26/32
103/103 [==============================] - 15s 146ms/step - loss: 0.6847 - recall: 0.6134 - recall_1: 0.5604 - precision: 0.5896 - precision_1: 0.5902 - val_loss: 0.6736 - val_recall: 0.5276 - val_recall_1: 0.6294 - val_precision: 0.5962 - val_precision_1: 0.5622
Epoch 00026: val_loss did not improve from 0.67161
Epoch 27/32
103/103 [==============================] - 15s 145ms/step - loss: 0.6924 - recall: 0.5688 - recall_1: 0.5704 - precision: 0.5746 - precision_1: 0.5649 - val_loss: 0.6697 - val_recall: 0.6283 - val_recall_1: 0.5373 - val_precision: 0.5848 - val_precision_1: 0.5822
Epoch 00027: val_loss improved from 0.67161 to 0.66968, saving model to /data/models/bebi205/linear
INFO:tensorflow:Assets written to: /data/models/bebi205/linear/assets
Epoch 28/32
103/103 [==============================] - 15s 145ms/step - loss: 0.6870 - recall: 0.5870 - recall_1: 0.5822 - precision: 0.5911 - precision_1: 0.5785 - val_loss: 0.7103 - val_recall: 0.8873 - val_recall_1: 0.2065 - val_precision: 0.5370 - val_precision_1: 0.6385
Epoch 00028: val_loss did not improve from 0.66968
Epoch 29/32
103/103 [==============================] - 15s 145ms/step - loss: 0.6907 - recall: 0.6234 - recall_1: 0.5596 - precision: 0.5903 - precision_1: 0.5966 - val_loss: 0.6807 - val_recall: 0.8561 - val_recall_1: 0.2910 - val_precision: 0.5561 - val_precision_1: 0.6610
Epoch 00029: val_loss did not improve from 0.66968
Epoch 30/32
103/103 [==============================] - 15s 144ms/step - loss: 0.6980 - recall: 0.5625 - recall_1: 0.5695 - precision: 0.5710 - precision_1: 0.5614 - val_loss: 0.6773 - val_recall: 0.7962 - val_recall_1: 0.3259 - val_precision: 0.5506 - val_precision_1: 0.6065
Epoch 00030: val_loss did not improve from 0.66968
Epoch 00030: ReduceLROnPlateau reducing learning rate to 7.812500371073838e-06.
Epoch 31/32
103/103 [==============================] - 15s 143ms/step - loss: 0.6846 - recall: 0.5425 - recall_1: 0.6250 - precision: 0.5963 - precision_1: 0.5726 - val_loss: 0.6667 - val_recall: 0.6835 - val_recall_1: 0.4627 - val_precision: 0.5689 - val_precision_1: 0.5849
Epoch 00031: val_loss improved from 0.66968 to 0.66671, saving model to /data/models/bebi205/linear
INFO:tensorflow:Assets written to: /data/models/bebi205/linear/assets
Epoch 32/32
103/103 [==============================] - 15s 144ms/step - loss: 0.6839 - recall: 0.5654 - recall_1: 0.5964 - precision: 0.5902 - precision_1: 0.5719 - val_loss: 0.6719 - val_recall: 0.7386 - val_recall_1: 0.3731 - val_precision: 0.5500 - val_precision_1: 0.5792
Epoch 00032: val_loss did not improve from 0.66671
<tensorflow.python.keras.callbacks.History at 0x7efa8e21e400>
# Train the fully connected neural network
fc_classifier.fit(db.train_dataset,
validation_data=db.val_dataset,
epochs=n_epochs,
verbose=1,
callbacks=fc_callbacks)
Epoch 1/32
103/103 [==============================] - 17s 149ms/step - loss: 0.8126 - recall: 0.6216 - recall_1: 0.4587 - precision: 0.5419 - precision_1: 0.5409 - val_loss: 2.2140 - val_recall: 0.0000e+00 - val_recall_1: 1.0000 - val_precision: 0.0000e+00 - val_precision_1: 0.4908
Epoch 00001: val_loss improved from inf to 2.21402, saving model to /data/models/bebi205/fc
INFO:tensorflow:Assets written to: /data/models/bebi205/fc/assets
Epoch 2/32
103/103 [==============================] - 15s 147ms/step - loss: 0.6989 - recall: 0.6168 - recall_1: 0.5339 - precision: 0.5758 - precision_1: 0.5760 - val_loss: 0.7440 - val_recall: 0.4700 - val_recall_1: 0.5945 - val_precision: 0.5460 - val_precision_1: 0.5196
Epoch 00002: val_loss improved from 2.21402 to 0.74405, saving model to /data/models/bebi205/fc
INFO:tensorflow:Assets written to: /data/models/bebi205/fc/assets
Epoch 3/32
103/103 [==============================] - 15s 146ms/step - loss: 0.6844 - recall: 0.6225 - recall_1: 0.5280 - precision: 0.5795 - precision_1: 0.5723 - val_loss: 0.8466 - val_recall: 0.0647 - val_recall_1: 0.9602 - val_precision: 0.6279 - val_precision_1: 0.4974
Epoch 00003: val_loss did not improve from 0.74405
Epoch 4/32
103/103 [==============================] - 15s 147ms/step - loss: 0.6753 - recall: 0.5899 - recall_1: 0.5828 - precision: 0.5905 - precision_1: 0.5824 - val_loss: 0.7350 - val_recall: 0.3621 - val_recall_1: 0.7463 - val_precision: 0.5968 - val_precision_1: 0.5300
Epoch 00004: val_loss improved from 0.74405 to 0.73501, saving model to /data/models/bebi205/fc
INFO:tensorflow:Assets written to: /data/models/bebi205/fc/assets
Epoch 5/32
103/103 [==============================] - 15s 147ms/step - loss: 0.6751 - recall: 0.6172 - recall_1: 0.5522 - precision: 0.5849 - precision_1: 0.5853 - val_loss: 0.7270 - val_recall: 0.7698 - val_recall_1: 0.3458 - val_precision: 0.5497 - val_precision_1: 0.5915
Epoch 00005: val_loss improved from 0.73501 to 0.72704, saving model to /data/models/bebi205/fc
INFO:tensorflow:Assets written to: /data/models/bebi205/fc/assets
Epoch 6/32
103/103 [==============================] - 15s 147ms/step - loss: 0.6730 - recall: 0.6011 - recall_1: 0.5751 - precision: 0.5926 - precision_1: 0.5838 - val_loss: 0.6948 - val_recall: 0.7986 - val_recall_1: 0.3259 - val_precision: 0.5513 - val_precision_1: 0.6093
Epoch 00006: val_loss improved from 0.72704 to 0.69481, saving model to /data/models/bebi205/fc
INFO:tensorflow:Assets written to: /data/models/bebi205/fc/assets
Epoch 7/32
103/103 [==============================] - 15s 146ms/step - loss: 0.6688 - recall: 0.5978 - recall_1: 0.5964 - precision: 0.5981 - precision_1: 0.5960 - val_loss: 0.6992 - val_recall: 0.7002 - val_recall_1: 0.4030 - val_precision: 0.5489 - val_precision_1: 0.5645
Epoch 00007: val_loss did not improve from 0.69481
Epoch 8/32
103/103 [==============================] - 15s 145ms/step - loss: 0.6679 - recall: 0.6125 - recall_1: 0.5752 - precision: 0.5951 - precision_1: 0.5928 - val_loss: 0.6687 - val_recall: 0.6499 - val_recall_1: 0.5124 - val_precision: 0.5803 - val_precision_1: 0.5852
Epoch 00008: val_loss improved from 0.69481 to 0.66869, saving model to /data/models/bebi205/fc
INFO:tensorflow:Assets written to: /data/models/bebi205/fc/assets
Epoch 9/32
103/103 [==============================] - 15s 146ms/step - loss: 0.6590 - recall: 0.6230 - recall_1: 0.5788 - precision: 0.6027 - precision_1: 0.5996 - val_loss: 0.6661 - val_recall: 0.6451 - val_recall_1: 0.5249 - val_precision: 0.5848 - val_precision_1: 0.5877
Epoch 00009: val_loss improved from 0.66869 to 0.66608, saving model to /data/models/bebi205/fc
INFO:tensorflow:Assets written to: /data/models/bebi205/fc/assets
Epoch 10/32
103/103 [==============================] - 15s 147ms/step - loss: 0.6665 - recall: 0.6039 - recall_1: 0.5812 - precision: 0.5961 - precision_1: 0.5891 - val_loss: 0.6764 - val_recall: 0.5468 - val_recall_1: 0.6070 - val_precision: 0.5907 - val_precision_1: 0.5635
Epoch 00010: val_loss did not improve from 0.66608
Epoch 11/32
103/103 [==============================] - 15s 147ms/step - loss: 0.6580 - recall: 0.6117 - recall_1: 0.5966 - precision: 0.6083 - precision_1: 0.6003 - val_loss: 0.6544 - val_recall: 0.7314 - val_recall_1: 0.4851 - val_precision: 0.5957 - val_precision_1: 0.6352
Epoch 00011: val_loss improved from 0.66608 to 0.65436, saving model to /data/models/bebi205/fc
INFO:tensorflow:Assets written to: /data/models/bebi205/fc/assets
Epoch 12/32
103/103 [==============================] - 15s 147ms/step - loss: 0.6574 - recall: 0.6392 - recall_1: 0.5573 - precision: 0.5952 - precision_1: 0.6028 - val_loss: 0.6639 - val_recall: 0.6835 - val_recall_1: 0.5000 - val_precision: 0.5864 - val_precision_1: 0.6036
Epoch 00012: val_loss did not improve from 0.65436
Epoch 13/32
103/103 [==============================] - 15s 146ms/step - loss: 0.6618 - recall: 0.6271 - recall_1: 0.5692 - precision: 0.5976 - precision_1: 0.5995 - val_loss: 0.6894 - val_recall: 0.8633 - val_recall_1: 0.2786 - val_precision: 0.5538 - val_precision_1: 0.6627
Epoch 00013: val_loss did not improve from 0.65436
Epoch 14/32
103/103 [==============================] - 15s 145ms/step - loss: 0.6587 - recall: 0.6162 - recall_1: 0.5883 - precision: 0.6037 - precision_1: 0.6009 - val_loss: 0.7292 - val_recall: 0.9209 - val_recall_1: 0.2139 - val_precision: 0.5486 - val_precision_1: 0.7227
Epoch 00014: val_loss did not improve from 0.65436
Epoch 00014: ReduceLROnPlateau reducing learning rate to 0.0005000000237487257.
Epoch 15/32
103/103 [==============================] - 15s 147ms/step - loss: 0.6536 - recall: 0.5975 - recall_1: 0.6208 - precision: 0.6168 - precision_1: 0.6018 - val_loss: 0.6768 - val_recall: 0.8345 - val_recall_1: 0.3308 - val_precision: 0.5640 - val_precision_1: 0.6584
Epoch 00015: val_loss did not improve from 0.65436
Epoch 16/32
103/103 [==============================] - 15s 145ms/step - loss: 0.6530 - recall: 0.6361 - recall_1: 0.5909 - precision: 0.6133 - precision_1: 0.6141 - val_loss: 0.7112 - val_recall: 0.8753 - val_recall_1: 0.2463 - val_precision: 0.5464 - val_precision_1: 0.6556
Epoch 00016: val_loss did not improve from 0.65436
Epoch 17/32
103/103 [==============================] - 15s 146ms/step - loss: 0.6536 - recall: 0.6093 - recall_1: 0.5964 - precision: 0.6078 - precision_1: 0.5979 - val_loss: 0.6723 - val_recall: 0.4868 - val_recall_1: 0.6940 - val_precision: 0.6227 - val_precision_1: 0.5659
Epoch 00017: val_loss did not improve from 0.65436
Epoch 00017: ReduceLROnPlateau reducing learning rate to 0.0002500000118743628.
Epoch 18/32
103/103 [==============================] - 15s 145ms/step - loss: 0.6508 - recall: 0.6058 - recall_1: 0.6085 - precision: 0.6119 - precision_1: 0.6024 - val_loss: 0.6821 - val_recall: 0.8465 - val_recall_1: 0.2960 - val_precision: 0.5550 - val_precision_1: 0.6503
Epoch 00018: val_loss did not improve from 0.65436
Epoch 19/32
103/103 [==============================] - 15s 147ms/step - loss: 0.6493 - recall: 0.6147 - recall_1: 0.5995 - precision: 0.6121 - precision_1: 0.6022 - val_loss: 0.6648 - val_recall: 0.8106 - val_recall_1: 0.3358 - val_precision: 0.5587 - val_precision_1: 0.6308
Epoch 00019: val_loss did not improve from 0.65436
Epoch 20/32
103/103 [==============================] - 15s 146ms/step - loss: 0.6563 - recall: 0.6079 - recall_1: 0.6014 - precision: 0.6122 - precision_1: 0.5971 - val_loss: 0.6761 - val_recall: 0.8321 - val_recall_1: 0.3159 - val_precision: 0.5579 - val_precision_1: 0.6447
Epoch 00020: val_loss did not improve from 0.65436
Epoch 00020: ReduceLROnPlateau reducing learning rate to 0.0001250000059371814.
Epoch 21/32
103/103 [==============================] - 15s 146ms/step - loss: 0.6471 - recall: 0.6082 - recall_1: 0.6219 - precision: 0.6216 - precision_1: 0.6084 - val_loss: 0.6808 - val_recall: 0.8513 - val_recall_1: 0.2985 - val_precision: 0.5573 - val_precision_1: 0.6593
Epoch 00021: val_loss did not improve from 0.65436
Epoch 22/32
103/103 [==============================] - 15s 148ms/step - loss: 0.6436 - recall: 0.6132 - recall_1: 0.6352 - precision: 0.6315 - precision_1: 0.6170 - val_loss: 0.6735 - val_recall: 0.8177 - val_recall_1: 0.3159 - val_precision: 0.5536 - val_precision_1: 0.6256
Epoch 00022: val_loss did not improve from 0.65436
Epoch 23/32
103/103 [==============================] - 15s 147ms/step - loss: 0.6481 - recall: 0.6044 - recall_1: 0.6279 - precision: 0.6246 - precision_1: 0.6077 - val_loss: 0.6736 - val_recall: 0.8369 - val_recall_1: 0.3259 - val_precision: 0.5629 - val_precision_1: 0.6583
Epoch 00023: val_loss did not improve from 0.65436
Epoch 00023: ReduceLROnPlateau reducing learning rate to 6.25000029685907e-05.
Epoch 24/32
103/103 [==============================] - 15s 146ms/step - loss: 0.6457 - recall: 0.6065 - recall_1: 0.6253 - precision: 0.6236 - precision_1: 0.6082 - val_loss: 0.6661 - val_recall: 0.8058 - val_recall_1: 0.3657 - val_precision: 0.5685 - val_precision_1: 0.6447
Epoch 00024: val_loss did not improve from 0.65436
Epoch 25/32
103/103 [==============================] - 15s 145ms/step - loss: 0.6432 - recall: 0.6107 - recall_1: 0.6325 - precision: 0.6288 - precision_1: 0.6145 - val_loss: 0.6725 - val_recall: 0.8297 - val_recall_1: 0.3308 - val_precision: 0.5626 - val_precision_1: 0.6520
Epoch 00025: val_loss did not improve from 0.65436
Epoch 26/32
103/103 [==============================] - 15s 145ms/step - loss: 0.6456 - recall: 0.6188 - recall_1: 0.6143 - precision: 0.6202 - precision_1: 0.6130 - val_loss: 0.6728 - val_recall: 0.8297 - val_recall_1: 0.3259 - val_precision: 0.5608 - val_precision_1: 0.6485
Epoch 00026: val_loss did not improve from 0.65436
Epoch 00026: ReduceLROnPlateau reducing learning rate to 3.125000148429535e-05.
Epoch 27/32
103/103 [==============================] - 15s 147ms/step - loss: 0.6452 - recall: 0.6163 - recall_1: 0.6114 - precision: 0.6159 - precision_1: 0.6118 - val_loss: 0.6731 - val_recall: 0.8321 - val_recall_1: 0.3308 - val_precision: 0.5633 - val_precision_1: 0.6552
Epoch 00027: val_loss did not improve from 0.65436
Epoch 28/32
103/103 [==============================] - 15s 143ms/step - loss: 0.6458 - recall: 0.6271 - recall_1: 0.6240 - precision: 0.6316 - precision_1: 0.6195 - val_loss: 0.6713 - val_recall: 0.8201 - val_recall_1: 0.3433 - val_precision: 0.5644 - val_precision_1: 0.6479
Epoch 00028: val_loss did not improve from 0.65436
Epoch 29/32
103/103 [==============================] - 15s 144ms/step - loss: 0.6450 - recall: 0.6145 - recall_1: 0.6312 - precision: 0.6309 - precision_1: 0.6148 - val_loss: 0.6663 - val_recall: 0.8153 - val_recall_1: 0.3483 - val_precision: 0.5648 - val_precision_1: 0.6452
Epoch 00029: val_loss did not improve from 0.65436
Epoch 00029: ReduceLROnPlateau reducing learning rate to 1.5625000742147677e-05.
Epoch 30/32
103/103 [==============================] - 15s 146ms/step - loss: 0.6395 - recall: 0.6385 - recall_1: 0.6254 - precision: 0.6347 - precision_1: 0.6294 - val_loss: 0.6674 - val_recall: 0.8201 - val_recall_1: 0.3433 - val_precision: 0.5644 - val_precision_1: 0.6479
Epoch 00030: val_loss did not improve from 0.65436
Epoch 31/32
103/103 [==============================] - 15s 144ms/step - loss: 0.6415 - recall: 0.6275 - recall_1: 0.6160 - precision: 0.6277 - precision_1: 0.6158 - val_loss: 0.6668 - val_recall: 0.8201 - val_recall_1: 0.3483 - val_precision: 0.5662 - val_precision_1: 0.6512
Epoch 00031: val_loss did not improve from 0.65436
Epoch 32/32
103/103 [==============================] - 15s 146ms/step - loss: 0.6428 - recall: 0.6176 - recall_1: 0.6209 - precision: 0.6260 - precision_1: 0.6125 - val_loss: 0.6676 - val_recall: 0.8177 - val_recall_1: 0.3483 - val_precision: 0.5655 - val_precision_1: 0.6481
Epoch 00032: val_loss did not improve from 0.65436
Epoch 00032: ReduceLROnPlateau reducing learning rate to 7.812500371073838e-06.
<tensorflow.python.keras.callbacks.History at 0x7efa8e26da58>
# Train the convolutional neural network
conv_classifier.fit(db.train_dataset,
validation_data=db.val_dataset,
epochs=n_epochs,
verbose=1,
callbacks=conv_callbacks)
Epoch 1/32
103/103 [==============================] - 17s 165ms/step - loss: 0.3076 - recall: 0.8679 - recall_1: 0.8671 - precision: 0.8705 - precision_1: 0.8645 - val_loss: 0.3842 - val_recall: 0.7973 - val_recall_1: 0.8619 - val_precision: 0.8263 - val_precision_1: 0.8377
Epoch 00001: val_loss improved from 0.38560 to 0.38423, saving model to /data/models/bebi205/conv
INFO:tensorflow:Assets written to: /data/models/bebi205/conv/assets
Epoch 2/32
103/103 [==============================] - 17s 170ms/step - loss: 0.2913 - recall: 0.8767 - recall_1: 0.8752 - precision: 0.8785 - precision_1: 0.8733 - val_loss: 0.4387 - val_recall: 0.7351 - val_recall_1: 0.8864 - val_precision: 0.8421 - val_precision_1: 0.8024
Epoch 00002: val_loss did not improve from 0.38423
Epoch 3/32
103/103 [==============================] - 17s 167ms/step - loss: 0.2866 - recall: 0.8761 - recall_1: 0.8712 - precision: 0.8750 - precision_1: 0.8722 - val_loss: 0.3759 - val_recall: 0.8216 - val_recall_1: 0.8641 - val_precision: 0.8329 - val_precision_1: 0.8546
Epoch 00003: val_loss improved from 0.38423 to 0.37594, saving model to /data/models/bebi205/conv
INFO:tensorflow:Assets written to: /data/models/bebi205/conv/assets
Epoch 4/32
103/103 [==============================] - 17s 167ms/step - loss: 0.2883 - recall: 0.8818 - recall_1: 0.8743 - precision: 0.8783 - precision_1: 0.8778 - val_loss: 0.4123 - val_recall: 0.7595 - val_recall_1: 0.8953 - val_precision: 0.8567 - val_precision_1: 0.8187
Epoch 00004: val_loss did not improve from 0.37594
Epoch 5/32
103/103 [==============================] - 17s 169ms/step - loss: 0.2891 - recall: 0.8791 - recall_1: 0.8687 - precision: 0.8733 - precision_1: 0.8746 - val_loss: 0.3799 - val_recall: 0.8486 - val_recall_1: 0.8575 - val_precision: 0.8307 - val_precision_1: 0.8730
Epoch 00005: val_loss did not improve from 0.37594
Epoch 6/32
103/103 [==============================] - 17s 169ms/step - loss: 0.2864 - recall: 0.8824 - recall_1: 0.8693 - precision: 0.8742 - precision_1: 0.8777 - val_loss: 0.3760 - val_recall: 0.8541 - val_recall_1: 0.8463 - val_precision: 0.8208 - val_precision_1: 0.8756
Epoch 00006: val_loss did not improve from 0.37594
Epoch 00006: ReduceLROnPlateau reducing learning rate to 3.125000148429535e-05.
Epoch 7/32
103/103 [==============================] - 18s 172ms/step - loss: 0.2877 - recall: 0.8809 - recall_1: 0.8746 - precision: 0.8785 - precision_1: 0.8770 - val_loss: 0.3616 - val_recall: 0.8432 - val_recall_1: 0.8597 - val_precision: 0.8320 - val_precision_1: 0.8694
Epoch 00007: val_loss improved from 0.37594 to 0.36159, saving model to /data/models/bebi205/conv
INFO:tensorflow:Assets written to: /data/models/bebi205/conv/assets
Epoch 8/32
103/103 [==============================] - 18s 171ms/step - loss: 0.2883 - recall: 0.8779 - recall_1: 0.8792 - precision: 0.8821 - precision_1: 0.8749 - val_loss: 0.3650 - val_recall: 0.8270 - val_recall_1: 0.8708 - val_precision: 0.8407 - val_precision_1: 0.8593
Epoch 00008: val_loss did not improve from 0.36159
Epoch 9/32
103/103 [==============================] - 17s 168ms/step - loss: 0.2799 - recall: 0.8854 - recall_1: 0.8792 - precision: 0.8830 - precision_1: 0.8817 - val_loss: 0.3776 - val_recall: 0.8000 - val_recall_1: 0.8909 - val_precision: 0.8580 - val_precision_1: 0.8439
Epoch 00009: val_loss did not improve from 0.36159
Epoch 10/32
103/103 [==============================] - 18s 170ms/step - loss: 0.2776 - recall: 0.8803 - recall_1: 0.8749 - precision: 0.8787 - precision_1: 0.8765 - val_loss: 0.3623 - val_recall: 0.8730 - val_recall_1: 0.8441 - val_precision: 0.8219 - val_precision_1: 0.8897
Epoch 00010: val_loss did not improve from 0.36159
Epoch 00010: ReduceLROnPlateau reducing learning rate to 1.5625000742147677e-05.
Epoch 11/32
103/103 [==============================] - 17s 169ms/step - loss: 0.2782 - recall: 0.8869 - recall_1: 0.8767 - precision: 0.8811 - precision_1: 0.8828 - val_loss: 0.3648 - val_recall: 0.8351 - val_recall_1: 0.8575 - val_precision: 0.8284 - val_precision_1: 0.8632
Epoch 00011: val_loss did not improve from 0.36159
Epoch 12/32
103/103 [==============================] - 17s 166ms/step - loss: 0.2789 - recall: 0.8863 - recall_1: 0.8749 - precision: 0.8794 - precision_1: 0.8820 - val_loss: 0.3640 - val_recall: 0.8297 - val_recall_1: 0.8686 - val_precision: 0.8388 - val_precision_1: 0.8609
Epoch 00012: val_loss did not improve from 0.36159
Epoch 13/32
103/103 [==============================] - 17s 169ms/step - loss: 0.2739 - recall: 0.8878 - recall_1: 0.8761 - precision: 0.8806 - precision_1: 0.8835 - val_loss: 0.3674 - val_recall: 0.8243 - val_recall_1: 0.8641 - val_precision: 0.8333 - val_precision_1: 0.8565
Epoch 00013: val_loss did not improve from 0.36159
Epoch 00013: ReduceLROnPlateau reducing learning rate to 7.812500371073838e-06.
Epoch 14/32
103/103 [==============================] - 17s 167ms/step - loss: 0.2768 - recall: 0.8860 - recall_1: 0.8854 - precision: 0.8884 - precision_1: 0.8830 - val_loss: 0.3738 - val_recall: 0.8135 - val_recall_1: 0.8731 - val_precision: 0.8408 - val_precision_1: 0.8503
Epoch 00014: val_loss did not improve from 0.36159
Epoch 15/32
103/103 [==============================] - 17s 169ms/step - loss: 0.2770 - recall: 0.8842 - recall_1: 0.8786 - precision: 0.8823 - precision_1: 0.8805 - val_loss: 0.3753 - val_recall: 0.8270 - val_recall_1: 0.8686 - val_precision: 0.8384 - val_precision_1: 0.8590
Epoch 00015: val_loss did not improve from 0.36159
Epoch 16/32
103/103 [==============================] - 18s 173ms/step - loss: 0.2710 - recall: 0.8824 - recall_1: 0.8823 - precision: 0.8853 - precision_1: 0.8793 - val_loss: 0.3682 - val_recall: 0.8351 - val_recall_1: 0.8575 - val_precision: 0.8284 - val_precision_1: 0.8632
Epoch 00016: val_loss did not improve from 0.36159
Epoch 00016: ReduceLROnPlateau reducing learning rate to 3.906250185536919e-06.
Epoch 17/32
103/103 [==============================] - 18s 174ms/step - loss: 0.2712 - recall: 0.8911 - recall_1: 0.8805 - precision: 0.8847 - precision_1: 0.8871 - val_loss: 0.3718 - val_recall: 0.8270 - val_recall_1: 0.8641 - val_precision: 0.8338 - val_precision_1: 0.8584
Epoch 00017: val_loss did not improve from 0.36159
Epoch 18/32
103/103 [==============================] - 18s 172ms/step - loss: 0.2754 - recall: 0.8812 - recall_1: 0.8798 - precision: 0.8830 - precision_1: 0.8779 - val_loss: 0.3728 - val_recall: 0.8270 - val_recall_1: 0.8641 - val_precision: 0.8338 - val_precision_1: 0.8584
Epoch 00018: val_loss did not improve from 0.36159
Epoch 19/32
103/103 [==============================] - 18s 174ms/step - loss: 0.2718 - recall: 0.8839 - recall_1: 0.8764 - precision: 0.8804 - precision_1: 0.8800 - val_loss: 0.3704 - val_recall: 0.8351 - val_recall_1: 0.8575 - val_precision: 0.8284 - val_precision_1: 0.8632
Epoch 00019: val_loss did not improve from 0.36159
Epoch 00019: ReduceLROnPlateau reducing learning rate to 1.9531250927684596e-06.
Epoch 20/32
103/103 [==============================] - 18s 174ms/step - loss: 0.2754 - recall: 0.8875 - recall_1: 0.8777 - precision: 0.8819 - precision_1: 0.8834 - val_loss: 0.3723 - val_recall: 0.8243 - val_recall_1: 0.8708 - val_precision: 0.8402 - val_precision_1: 0.8575
Epoch 00020: val_loss did not improve from 0.36159
Epoch 21/32
103/103 [==============================] - 18s 174ms/step - loss: 0.2672 - recall: 0.8917 - recall_1: 0.8817 - precision: 0.8858 - precision_1: 0.8877 - val_loss: 0.3736 - val_recall: 0.8243 - val_recall_1: 0.8686 - val_precision: 0.8379 - val_precision_1: 0.8571
Epoch 00021: val_loss did not improve from 0.36159
Epoch 22/32
103/103 [==============================] - 18s 176ms/step - loss: 0.2761 - recall: 0.8866 - recall_1: 0.8736 - precision: 0.8784 - precision_1: 0.8821 - val_loss: 0.3723 - val_recall: 0.8297 - val_recall_1: 0.8708 - val_precision: 0.8411 - val_precision_1: 0.8612
Epoch 00022: val_loss did not improve from 0.36159
Epoch 00022: ReduceLROnPlateau reducing learning rate to 9.765625463842298e-07.
Epoch 23/32
103/103 [==============================] - 18s 177ms/step - loss: 0.2741 - recall: 0.8833 - recall_1: 0.8814 - precision: 0.8846 - precision_1: 0.8800 - val_loss: 0.3729 - val_recall: 0.8270 - val_recall_1: 0.8686 - val_precision: 0.8384 - val_precision_1: 0.8590
Epoch 00023: val_loss did not improve from 0.36159
Epoch 24/32
103/103 [==============================] - 18s 174ms/step - loss: 0.2714 - recall: 0.8857 - recall_1: 0.8730 - precision: 0.8778 - precision_1: 0.8812 - val_loss: 0.3709 - val_recall: 0.8297 - val_recall_1: 0.8641 - val_precision: 0.8342 - val_precision_1: 0.8603
Epoch 00024: val_loss did not improve from 0.36159
Epoch 25/32
103/103 [==============================] - 18s 177ms/step - loss: 0.2714 - recall: 0.8884 - recall_1: 0.8857 - precision: 0.8889 - precision_1: 0.8852 - val_loss: 0.3715 - val_recall: 0.8297 - val_recall_1: 0.8641 - val_precision: 0.8342 - val_precision_1: 0.8603
Epoch 00025: val_loss did not improve from 0.36159
Epoch 00025: ReduceLROnPlateau reducing learning rate to 4.882812731921149e-07.
Epoch 26/32
103/103 [==============================] - 18s 175ms/step - loss: 0.2735 - recall: 0.8974 - recall_1: 0.8721 - precision: 0.8784 - precision_1: 0.8920 - val_loss: 0.3711 - val_recall: 0.8270 - val_recall_1: 0.8664 - val_precision: 0.8361 - val_precision_1: 0.8587
Epoch 00026: val_loss did not improve from 0.36159
Epoch 27/32
103/103 [==============================] - 18s 175ms/step - loss: 0.2747 - recall: 0.8812 - recall_1: 0.8823 - precision: 0.8852 - precision_1: 0.8782 - val_loss: 0.3739 - val_recall: 0.8270 - val_recall_1: 0.8686 - val_precision: 0.8384 - val_precision_1: 0.8590
Epoch 00027: val_loss did not improve from 0.36159
Epoch 28/32
103/103 [==============================] - 18s 175ms/step - loss: 0.2735 - recall: 0.8818 - recall_1: 0.8826 - precision: 0.8855 - precision_1: 0.8788 - val_loss: 0.3712 - val_recall: 0.8297 - val_recall_1: 0.8619 - val_precision: 0.8320 - val_precision_1: 0.8600
Epoch 00028: val_loss did not improve from 0.36159
Epoch 00028: ReduceLROnPlateau reducing learning rate to 2.4414063659605745e-07.
Epoch 29/32
103/103 [==============================] - 18s 177ms/step - loss: 0.2813 - recall: 0.8830 - recall_1: 0.8764 - precision: 0.8803 - precision_1: 0.8792 - val_loss: 0.3710 - val_recall: 0.8297 - val_recall_1: 0.8641 - val_precision: 0.8342 - val_precision_1: 0.8603
Epoch 00029: val_loss did not improve from 0.36159
Epoch 30/32
103/103 [==============================] - 18s 176ms/step - loss: 0.2740 - recall: 0.8851 - recall_1: 0.8808 - precision: 0.8843 - precision_1: 0.8816 - val_loss: 0.3720 - val_recall: 0.8270 - val_recall_1: 0.8641 - val_precision: 0.8338 - val_precision_1: 0.8584
Epoch 00030: val_loss did not improve from 0.36159
Epoch 31/32
103/103 [==============================] - 18s 176ms/step - loss: 0.2706 - recall: 0.8884 - recall_1: 0.8842 - precision: 0.8876 - precision_1: 0.8850 - val_loss: 0.3724 - val_recall: 0.8270 - val_recall_1: 0.8686 - val_precision: 0.8384 - val_precision_1: 0.8590
Epoch 00031: val_loss did not improve from 0.36159
Epoch 00031: ReduceLROnPlateau reducing learning rate to 1.2207031829802872e-07.
Epoch 32/32
103/103 [==============================] - 19s 182ms/step - loss: 0.2698 - recall: 0.8893 - recall_1: 0.8764 - precision: 0.8811 - precision_1: 0.8849 - val_loss: 0.3729 - val_recall: 0.8270 - val_recall_1: 0.8664 - val_precision: 0.8361 - val_precision_1: 0.8587
Epoch 00032: val_loss did not improve from 0.36159
<tensorflow.python.keras.callbacks.History at 0x7efc9c5dddd8>
Benchmark the model#
In this section, we will benchmark each model to assess the performance.
# Visualize some predictions
it = db.test_dataset.as_numpy_iterator()
fig, axes = plt.subplots(4, 2, figsize=(20,20))
for i in range(8):
X_test, y_test = it.next()
# Get an example image
X_sample = X_test[[i],...]
# Predict the label
y_pred_linear = linear_classifier.predict(X_sample)
y_pred_fc = fc_classifier.predict(X_sample)
y_pred_conv = conv_classifier.predict(X_sample)
# Display results
axes.flatten()[i].imshow(X_sample[0])
axes.flatten()[i].set_title('Label ' + str(y[i]) +', Conv prediction ' + str(y_pred_conv))
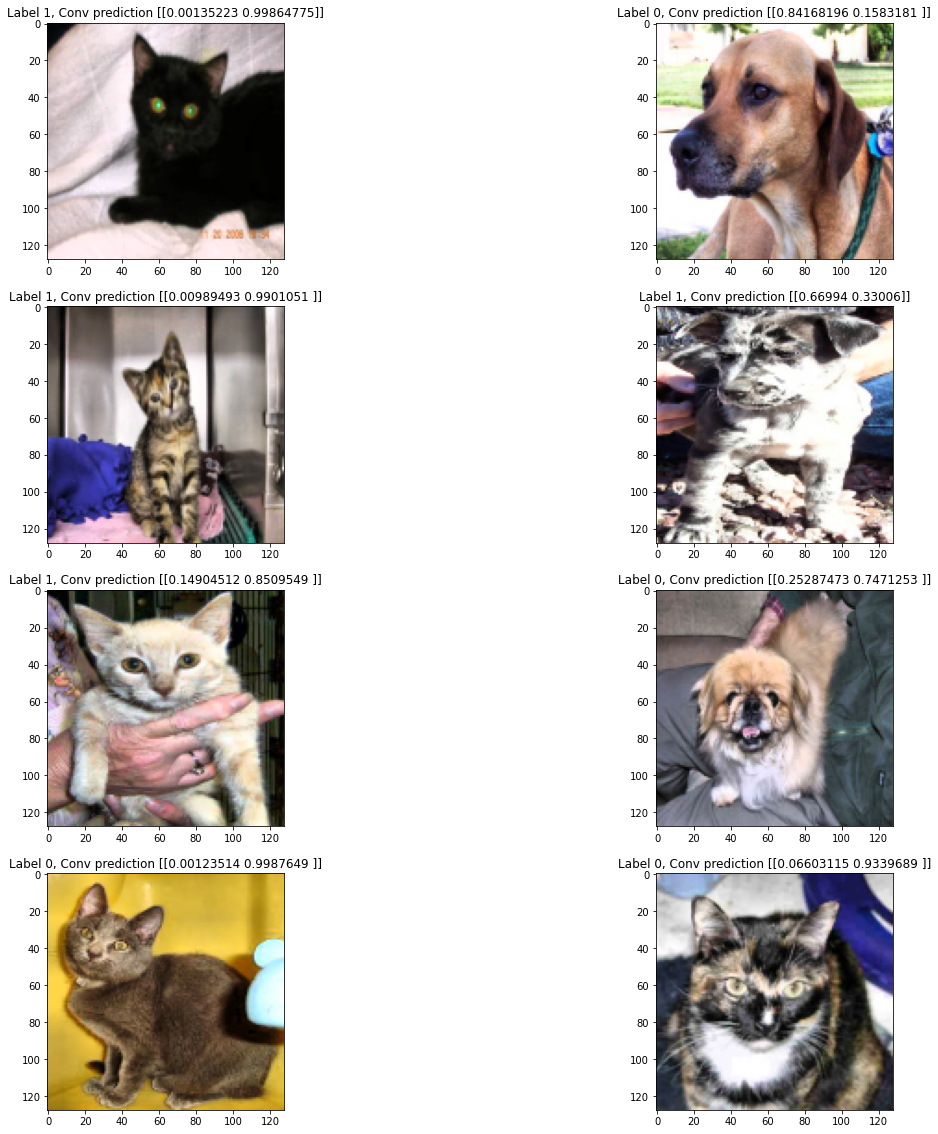
# Generate predictions
test_list = list(db.test_dataset.as_numpy_iterator())
X_test = [item[0] for item in test_list]
y_test = [item[1] for item in test_list]
X_test = np.concatenate(X_test, axis=0)
y_test = np.concatenate(y_test, axis=0)
y_test = np.argmax(y_test, axis=-1)
# Compute linear classifier metrics
y_pred = linear_classifier.predict(X_test)
y_pred = np.argmax(y_pred, axis=-1)
recall = sklearn.metrics.recall_score(y_test, y_pred)
precision = sklearn.metrics.precision_score(y_test, y_pred)
f1 = sklearn.metrics.f1_score(y_test, y_pred)
print('Linear Recall: {}'.format(recall))
print('Linear Precision: {}'.format(precision))
print('Linear F1 Score: {}'.format(f1))
# Compute conv metrics
y_pred = fc_classifier.predict(X_test)
y_pred = np.argmax(y_pred, axis=-1)
recall = sklearn.metrics.recall_score(y_test, y_pred)
precision = sklearn.metrics.precision_score(y_test, y_pred)
f1 = sklearn.metrics.f1_score(y_test, y_pred)
print('Fully Connected Recall: {}'.format(recall))
print('Fully Connected Precision: {}'.format(precision))
print('Fully Connected F1 Score: {}'.format(f1))
# Compute conv metrics
y_pred = conv_classifier.predict(X_test)
y_pred = np.argmax(y_pred, axis=-1)
recall = sklearn.metrics.recall_score(y_test, y_pred)
precision = sklearn.metrics.precision_score(y_test, y_pred)
f1 = sklearn.metrics.f1_score(y_test, y_pred)
print('Conv Recall: {}'.format(recall))
print('Conv Precision: {}'.format(precision))
print('Conv F1 Score: {}'.format(f1))
Linear Recall: 0.3492822966507177
Linear Precision: 0.6375545851528385
Linear F1 Score: 0.45131375579598143
Fully Connected Recall: 0.35406698564593303
Fully Connected Precision: 0.6851851851851852
Fully Connected F1 Score: 0.4668769716088329
Conv Recall: 0.8899521531100478
Conv Precision: 0.8493150684931506
Conv F1 Score: 0.869158878504673
%load_ext watermark
%watermark -u -d -vm --iversions
Last updated: 2021-04-28
Python implementation: CPython
Python version : 3.7.10
IPython version : 5.5.0
Compiler : GCC 7.5.0
OS : Linux
Release : 4.19.112+
Machine : x86_64
Processor : x86_64
CPU cores : 2
Architecture: 64bit
numpy : 1.19.5
imageio : 2.4.1
skimage : 0.16.2
matplotlib : 3.2.2
tensorflow : 2.4.1
tensorflow_addons: 0.12.1
sklearn : 0.0
pandas : 1.1.5
IPython : 5.5.0